If you don’t remember The Little Red Hen, a chicken found grain and asked the other farm animals to help throughout the journey of processing that grain. The hen asked if they would plant the seeds, help with the harvest, make the flour, and finally bake the bread, but the other animals refused to help until they could smell the goodness in the oven. At that point, they were more than happy to eat the bread. After 10+ years of architecting Data Governance Programs from scratch through maturity, I have found a lot of similarities to this fable.
TL;DR
A classic American fable is used to explain the resourcing constraints that hinder the establishment of Data Governance Programs before the use of Artificial Intelligence (AI) to automate Data Stewardship with crowd-sourced influences. Below are the parallels:
- Use AI to Grow a Glossary – Unstructured data assets are rich with content that should be leveraged to construct a business glossary. A glossary is a collection of concepts that already exist in unstructured data sources, like seeds of grain ready to be planted.
- Gather Information from AI Data Stewards – Organic material needs water and sunshine before baseline measurements are useful to determine growth rate. Likewise, the content curated by the AI and SME partnership will require care and feeding with the support of ontology experts to maximize the metadata available to curate data and measure success.
- Savor the Automation – The recipe for successful Data Governance calls for transparent accountability. Although crowd-sourcing methods can be leveraged to make recommendations, a uniform domain structure is ideal for identifying the right individuals to manage escalations across the enterprise. AI can lighten the load, but SMEs must be involved in every phase of execution.
- Indulge in Data with Sustainable Quality – Any good chef knows the final part in the cooking process is plating the meal. Presenting Data Governance with AI Stewardship will require a solution, framework, and role-based business process to make this effort consumable for crowd-sourced input.
Looking at the Data Governance (DG) process from beginning to end, leaders want the end-product that quality data brings to the organization. Most will agree transparent information ownership, trusted data sources with quality metrics, and unprecedented data literacy across the enterprise are characteristics of a successful program. However, even with funding and executive support, these programs can fail if the organization’s culture is not motivated to change. For most mid-size organizations in regulated industries, successful data governance may require a dozen full-time Data Stewards or a few dozen part-time individuals to reach their goals. It’s the investment in business stakeholder SME time that limits the successful launch of most DG programs.
Like The Little Red Hen, Data Literacy is a process that can be followed with fewer resources if AI and crowdsourcing aligns with your corporate values. Once lessons have been learned from AI implementation, the iterative refinement of data management is easier for contributors. Trust others will support the program after the initial investment shows an initial impact.
Use AI to Grow a Glossary
At this point, AI has made its way into every data conversation, and quality data is essential for AI models. Before investments are made in AI, data quality with curated data must be part of a practice, and not a stand-alone exercise before each AI model is developed. As an experienced Data Governance Manager responsible for designing and implementing these programs, the Stewardship role is vital for establishing criteria for Data Quality. To pull data from multiple sources, the data quality is only as good as its weakest link, and starting with a well-defined, enterprise-wide glossary is key.
While this may seem like a simple task, agreeing to the definition of a concept like Net Sales or Gross Profit can take more time than you think. Fortunately for Data Governance Programs, programs can shift to using AI tools to define terms and make recommendations, meaning the manual efforts of Data Stewards are greatly reduced. These AI provided definitions can be reinforced by crowdsourcing or corrected by Subject Matter Experts (SMEs). It may not seem like a game changer, but these enhancements nearly nullify the resource time required to create a glossary.
Curation of information is now AI Data Stewardship enabled by scanning, indexing and contextualizing organizational resources. The output is a machine-augmented business glossary that leverages AI Data Stewards that offer high productivity and integrates SME accuracy. Thankfully, end-users of governance tools can recommend modifications and initiate process flows that engage SMEs for selective curation efforts.
Gather Information from AI Data Stewards
Data Stewardship is a process followed by Subject Matter Experts to curate data and information. AI Data Stewards are technically designed, supportive functions that can be implemented to reduce the manual effort of Data Stewards. Previously, Data Stewards were trained to draft unfamiliar business terms using Google. With AI, a glossary is created by scanning unstructured data to identify Critical Data Elements (CDEs) and domains unique to your organization. To unlock the value of existing information, ontology experts customize Knowledge Graphs to interpret data relationships unveiling concepts that are statistically similar to each other. By combining related concepts together and analyzing term frequency and folder sprawling, the critical data elements for an organization are A.I.-identified. With the added support of Large Language Models (LLM), new A.I.-identified terms can be defined in a business glossary as part of a formal Data Management program.
Knowledge Graphs alone do not improve the quality of your data. However, formal Data Management programs, combined with best practices, can improve data quality by monitoring and alerting when data needs to be cleansed. AI Data Stewardship is at the intersection between Knowledge Graphs and Business Glossaries. From a user experience perspective, Knowledge Graphs allow for the representation of complex relationships between different pieces of data to be visually intuitive. More importantly, this partnership connects data literacy to the compliance adherence components of Data Management programs. Reducing risk and limiting data loss is a form of information monetization. The costly consequences from a lack of sensitive data protection should not be ignored while increasing data literacy.
Data Management Capabilities can have program level KPIs based on characteristics (e.g., primary data match rules, quality business rules, identity access management, and retention schedules) that quantifiably builds trust in your data. The quantity of implemented solutions (e.g., data lineage confirmations, quality threshold verification, and certified reusable datasets) can be measured for maturing programs. These metrics are foundational for managing escalations from crowd-sourced validations.
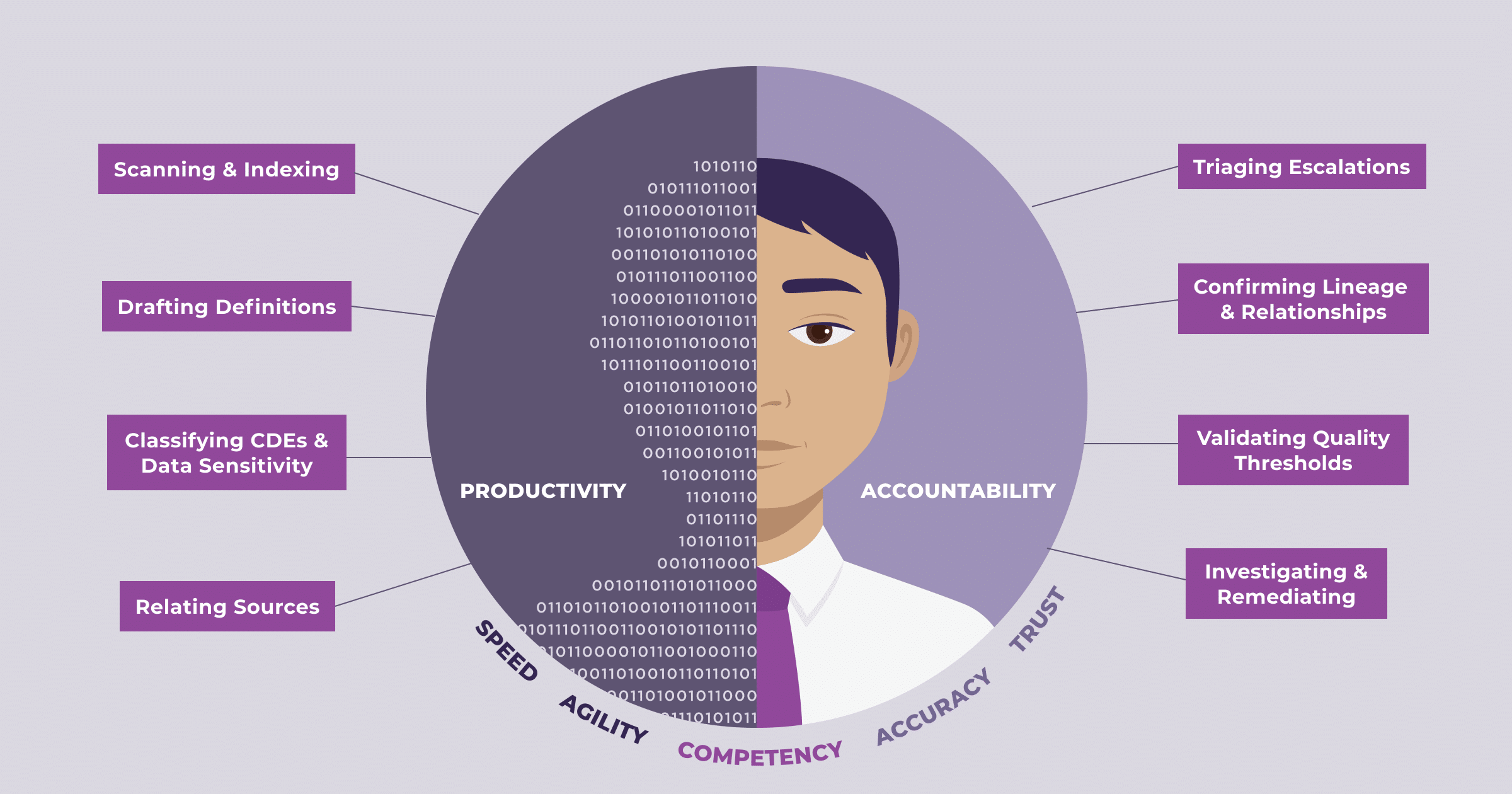
Savor the Automation
When the other farm animals in The Little Red Hen were asked to help, they exclaimed “Not I,” much like leaders being asked to allocate manual resources to a new Data Governance Program. Instead of rolling out the DG Program by domains or working groups, AI Data Stewards can execute across the enterprise simultaneously, accelerating what was previously a time-intensive process required before seeing DG payoff. It is like having a fresh bread smell pumping through your HVAC. Once leaders get a whiff of what AI Data Stewards can do, they will commit with “Oh, I will” let my team validate the AI recommendations. Let’s discuss how.
EK’s machine-augmented business glossary generation process scans unstructured data stores and uses Topic Modeling to rank the frequency of term usage and the count of root folders used in the organization. To enrich the indexing of terms, EK offers a predefined, industry-agnostic subdomain catalog (parent) with glossary terms (child) to relate new terms. As the business glossary grows, terms found in automated scans without robust definitions can leverage Large Language Models (LLM) and implied metadata attributes from the subdomains to fill in gaps in term definitions and attributes. The resulting product is a well-defined business glossary that defines critical information across every data domain.
As the terms are defined, their authoritative sources can be identified using AI support. Table usage statistics can be leveraged to speed up the curation process. To prevent AI hallucinations or pitfalls, human-in-the-loop processes can be used to allow Data Stewards to review and approve AI recommendations. Using characteristics from the underlying database (e.g., frequency of joins and usage statistics), AI can make very good inferences as to which data elements may require human review, initiating the data stewardship process. Enabling Business SMEs to correct, escalate or validate the AI Data Steward recommendations can reduce the manual process for curating data by 60%.
Indulge in Data with Sustainable Quality
Crowd-sourced curation of information starts with AI Data Stewardship. By engaging EK’s Advanced Data Management team with enterprise AI experts complemented by Knowledge Graph designers, your DG Program can baseline and collect operational metrics in a couple weeks instead of several months or years. Multiple accelerators, from prebuilt glossaries and domain catalogs to configurable frameworks and operating models, are ready for AI integration to improve the quality of information in any organization.
By considering these elements, businesses can efficiently build AI enabled Data Stewardship that empowers them to reap the benefits of SME curated data quality.
Does your organization need support building or improving your business glossary with AI? Contact us at info@enterprise-knowledge.com.