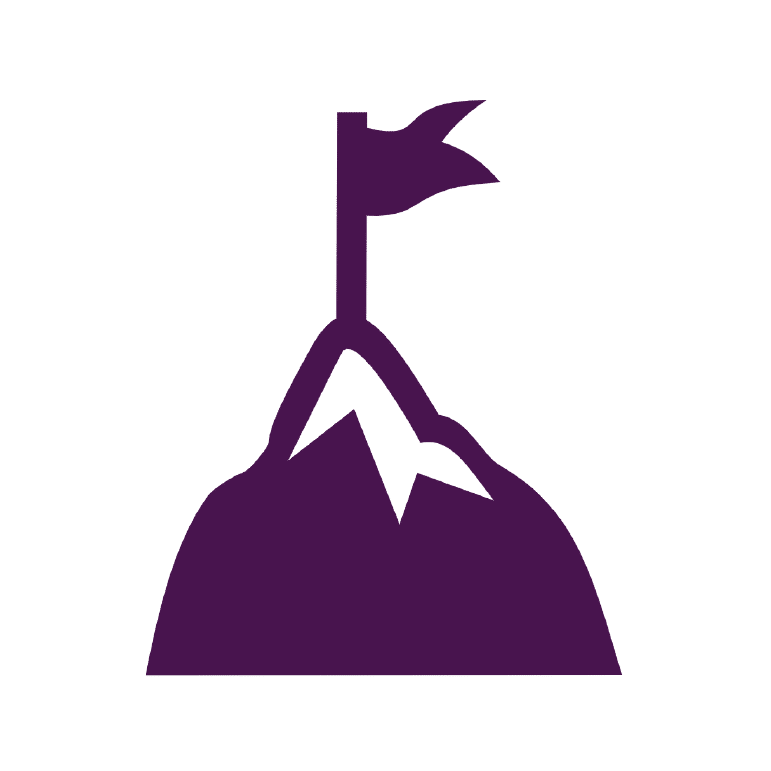
The Challenge
A multinational financial institution with a century-long legacy, celebrated for pioneering financial solutions and shaping the global economic landscape, relied on unstructured data for risk management. With a vast array of risks to consider, and a wealth of insights generated by risk analysts, relying on text-based risk descriptions that proved to be limited in creating consistent reporting and tracking of risk over time.
The risk analysis process consisted of risk assessors, employing natural language to articulate risks as they were identified and reported. However, due to the diverse vocabularies and writing styles prevalent across various departments and geographies, the utilization of these risk descriptions posed a considerable challenge in reusability and making them machine readable. Even when integrated with existing taxonomies, the varying language hindered the efficient aggregation and analysis of non-financial risks on a global scale. Using different vocabularies and taxonomies led to information silos, a lack of shared understanding across departments and products, and an overallocation of resources to analyze and extract valuable conclusions from texts that seemed to be very different.
The firm recognized the need for a more structured and uniform approach to risk assessment. The solution required the implementation of a new taxonomy and risk classification system to improve risk management practices, enhance decision-making, and optimize resource allocation. The new taxonomy and risk classification system would give the company a holistic view of risks and facilitate data-driven strategic decisions.
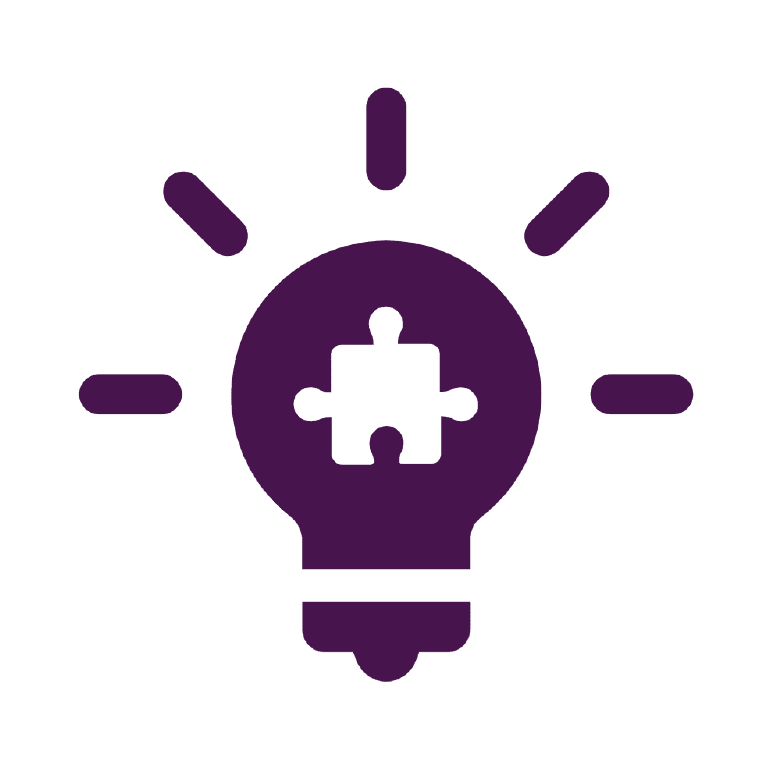
The Solution
To address this challenge, EK leveraged an agile process using generative AI (Gen AI) to expand and refine the client’s risk taxonomy. The goal was to move beyond free-text descriptions, enabling the financial institution to aggregate risks accurately for analytical purposes and ensure a comprehensive overview of their non-financial risk landscape. The solution contained the following components:
- Topic Modeling: Using a large language model (LLM) to create the text embeddings, EK implemented a semi-supervised clustering algorithm to group text descriptions based on semantic similarity. Each cluster included a set of representative texts and a set of keywords that best represented the text-based descriptions contained in the cluster. This component helped subject matter experts (SMEs) identify common themes and patterns within a large volume of non-financial risk data.
- Generative AI & Prompt Engineering: Risk experts offered specific instances of risk topics drawn from the themes identified in the clusters. These examples were then used in engineering a prompt to guide the language model in generating risk topics for all clusters. Adhering to the provided examples and cluster properties yielded a controlled vocabulary of risk topics that closely resembled the SMEs’ output but reflected the clusters’ contents.
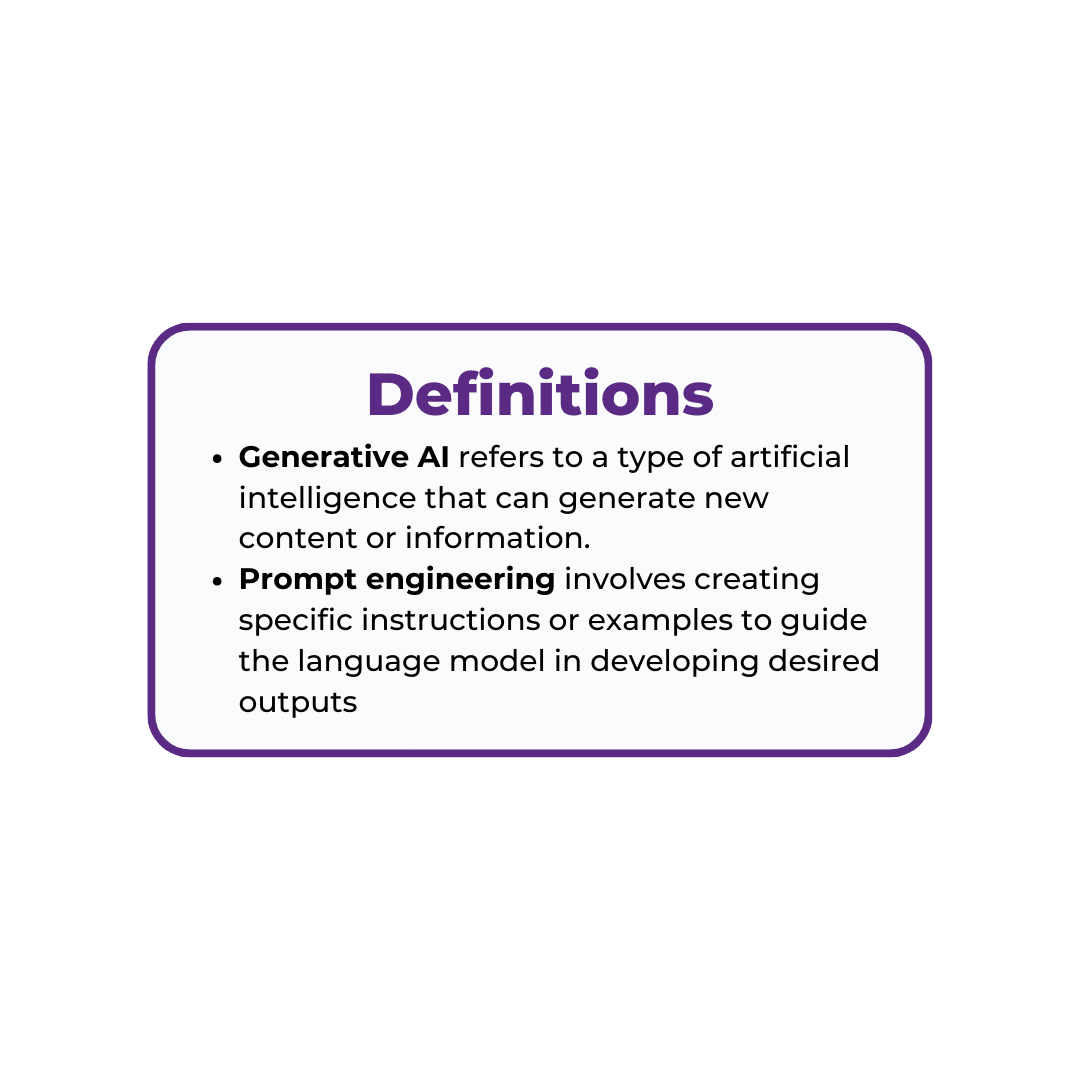
- Human-in-the-Loop Taxonomy Development: Given the nuanced and regulatory nature of risk management, it was critical to have human experts ultimately own the risk taxonomy rather than using the output of GenAI at face value. Deploying a similar process to Reinforcement Learning from Human Feedback (RLHF), human experts significantly improved the model-generated risk clusters and topics. The continued model fine-tuning facilitated risk experts to review, edit, create and approve risk topics at scale by providing a more efficient and comprehensive way of describing risks. SME review was then used to retrain the clustering algorithm, initiating a new cycle with more training data. The feedback loop between the AI model and human experts led to a final SME-approved taxonomy that accurately described the complexity of risk data in a way that could be used for large-scale risk events in the past and future.
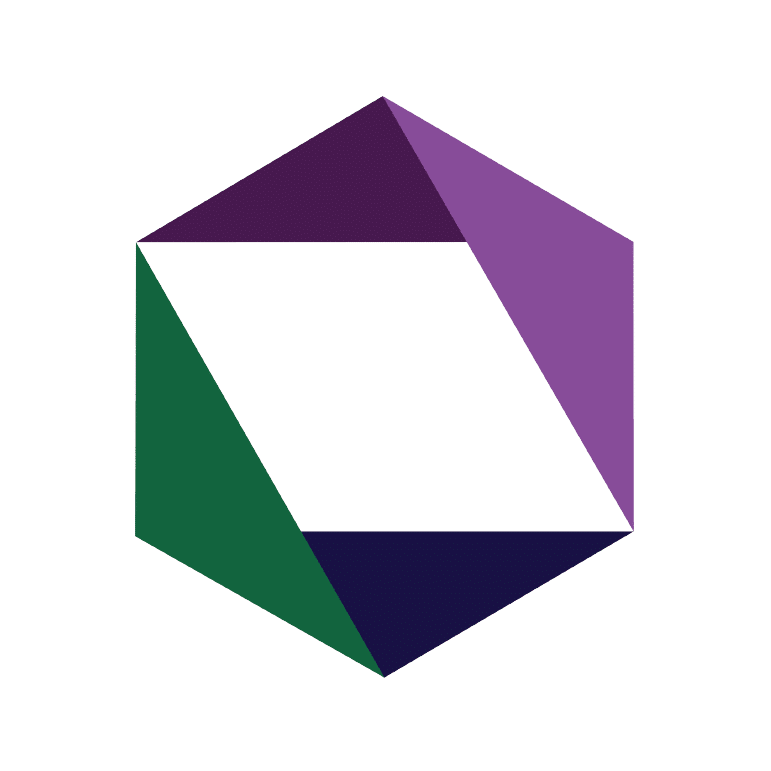
The EK Difference
The AI-assisted taxonomy development is one component of EK’s comprehensive transformation and enhancement of the risk data management environment for the firm. Bringing unparalleled expertise to the program, our team plays a pivotal role in guiding the transformation of risk data from current to future states. By providing strategic insights into data management, semantic technologies, AI models, and architecture development, we lay the foundation for creating semantic models that drive risk-reporting technology within the program.
One of our distinctive strengths lies in the consistent advisory and expert guidance we provide in semantic modeling for risks. This includes case-driven ontology modeling, enabling semi-automated and semantically enhanced risk assessment. Our quarterly releases of evolving semantic structures and enabling technologies align with the dynamic goals of the program, ensuring it stays at the forefront of technological advancements and risk management best practices. Simultaneously, our team offers architectural recommendations to connect, mature, and integrate technologies, fostering a robust future-state ecosystem.
Furthermore, the EK team leads in blending the firm’s diverse taxonomies and controlled vocabularies related to risk assessment. Our role is to lead forward-thinking and advanced data science techniques to move the program forward and continuously improve the firm’s processes at scale. We focus on creating a unified model from dozens of siloed vocabularies, ensuring streamlined and consistent reporting capabilities. Retaining historical mappings is crucial, allowing us to provide stakeholders with historical metrics and consistency in risk reporting processes. As an innovator in risk management, the EK team leverages advanced data science techniques, as exemplified by our engagement, employing semantic similarity algorithms and large language models to optimize the program’s future state of risk categories.
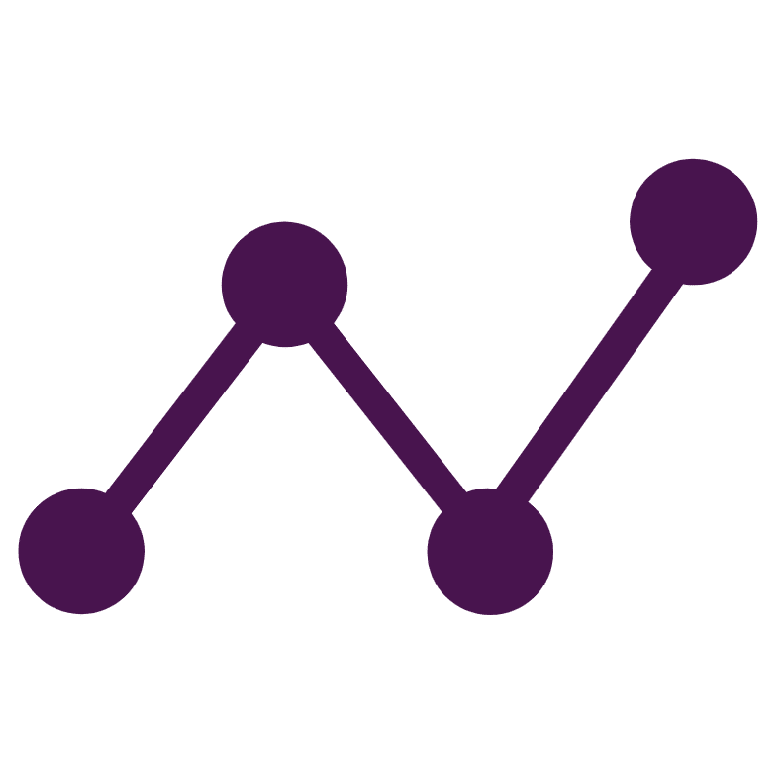
The Results
Leveraging an agile process, EK employed generative AI to expand and refine the risk taxonomy. This process involved a multi-faceted approach, incorporating topic modeling, generative AI, and prompt engineering. The outcome was a final taxonomy that offered a profound understanding of the interconnectedness of risks over time and across divisions, allowing the firm to assess better their potential impact on the organization on a global scale.
The human-in-the-loop taxonomy development further elevated the model-generated risk topics through expert reviews and categorizations. This process enabled the aggregation of about 10,000 yearly records into approximately 1,500 categories from the newly developed risk taxonomy. This aggregation reduced the time it took risk SMEs to review and categorize risk incidents from 45-50 minutes to 1-2 minutes per risk occurrence.
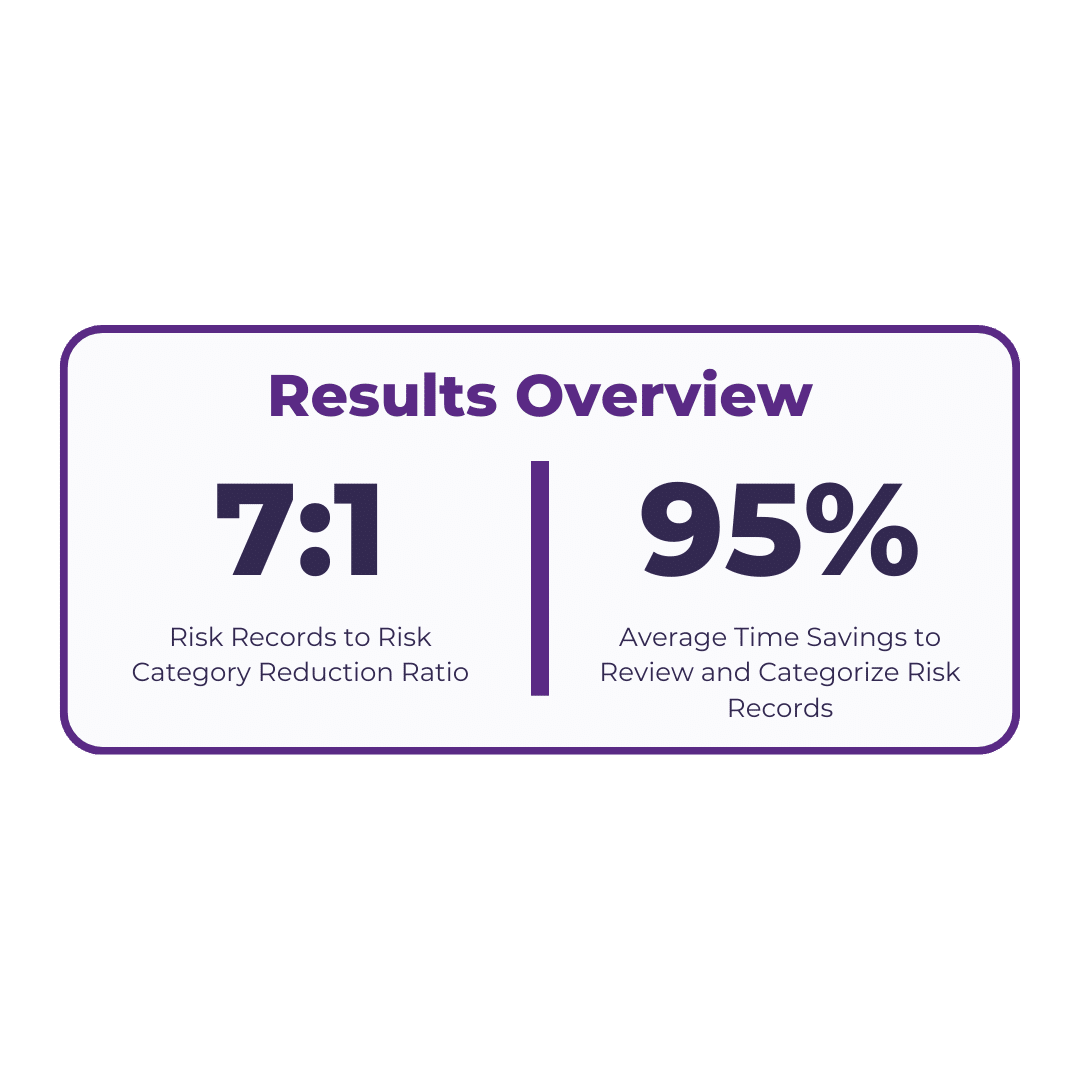
At EK, we specialize in transforming complex challenges into streamlined processes. By leveraging Generative AI, we revolutionized our client’s risk management use case, automating taxonomy development processes and providing an understanding of interconnected risks. Our demonstrated approach, combining advanced technology with human expertise through human-in-the-loop models, ensures not just automation but optimization, allowing your team to focus on strategic decision-making. Contact us for generative AI applications that can support your organization in handling your data complexities more efficiently and with improved foresight.