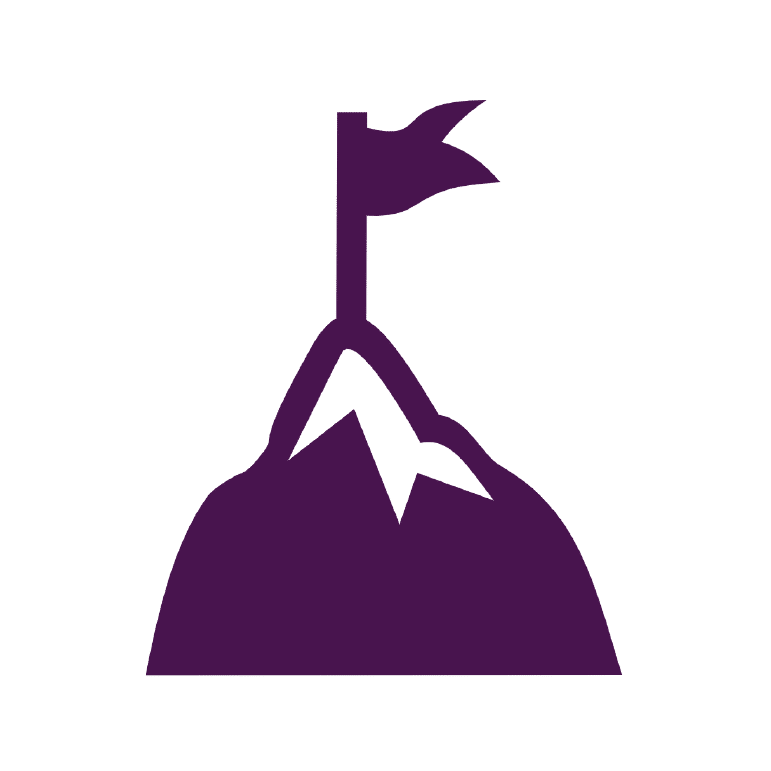
The Challenge
One of the largest global supply chain companies needed to provide its business users and leadership with a way to directly access and glean quick insights from their large and disparate data sources while using natural language search. They also wanted to ensure that their data analysts have the tools and processes available to manage and analyze this data. The data sets were stored in a large RDBMS data warehouse with little to no context attached, making it difficult to gauge their value, understand which information to use, and what questions that data could answer. The organization wanted to bring meaningful information and facts together to overcome these challenges and to make more timely and informed funding and investment decisions.
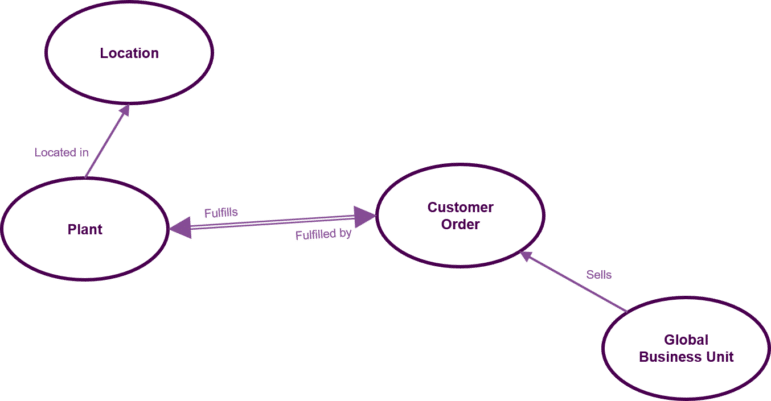
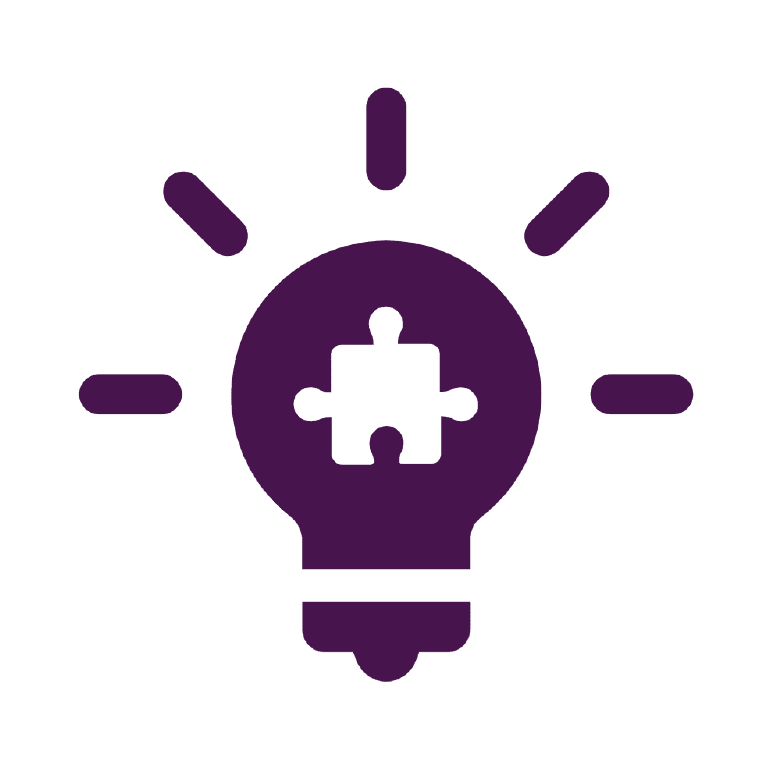
The Solution
By extracting key entities or metadata fields, such as topic, place, person, customer, plant, etc. from their sample files and data sets, Enterprise Knowledge (EK) developed an ontology to describe the key questions business users were interested in and how they, and their answers, relate to each other. EK then mapped the various data sets to the ontology and a knowledge graph, and leveraged semantic Natural Language Processing (NLP) capabilities to recognize user intent, link concepts, and dynamically generate the data queries that provide the response.
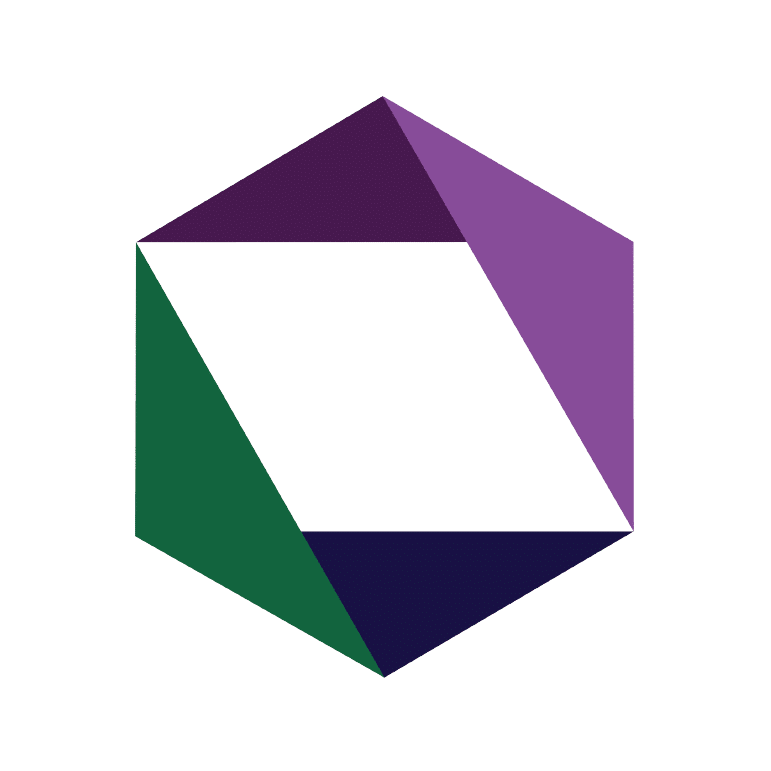
The EK Difference
Our experts worked closely with the organization’s own Data subject matter experts (SMEs) throughout the endeavor. We facilitated knowledge transfers and design sessions in order to refine use cases and to reach a clear definition of key information entities, and their relationships to each other, and to unleash the value of data contexts and meaning to the business. We then leveraged our data science expertise and efficient data Extract, Transform, and Load (ETL) logic to drive a rapid alignment of data elements with the natural language structure of English questions to identify user intent. Simultaneously, EK also leveraged a semantic data layer, allowing for the flexible mapping of disparate data source schemas into a single, unified data model that is easily digestible and accessible to both technical and nontechnical users.
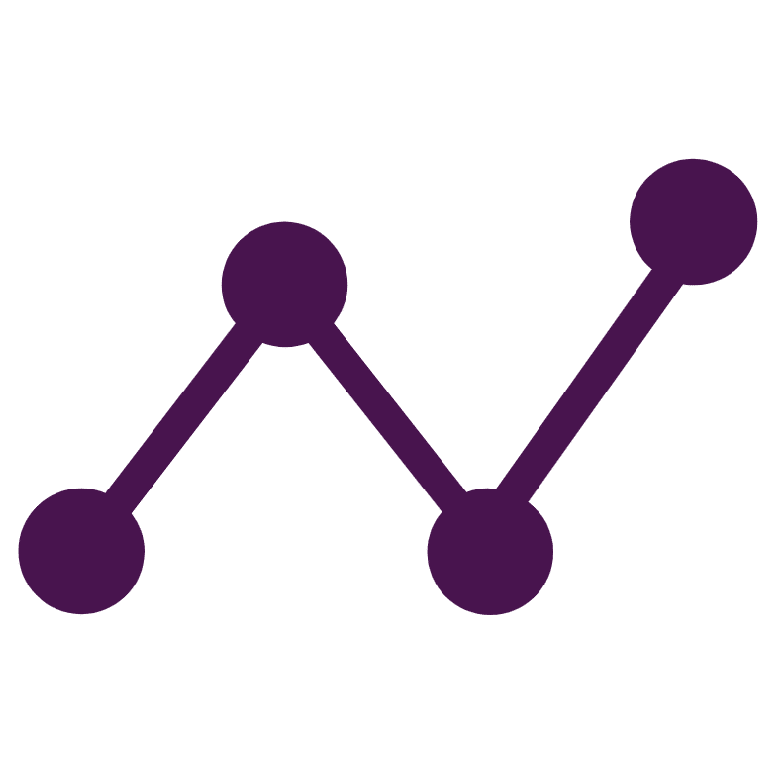
The Results
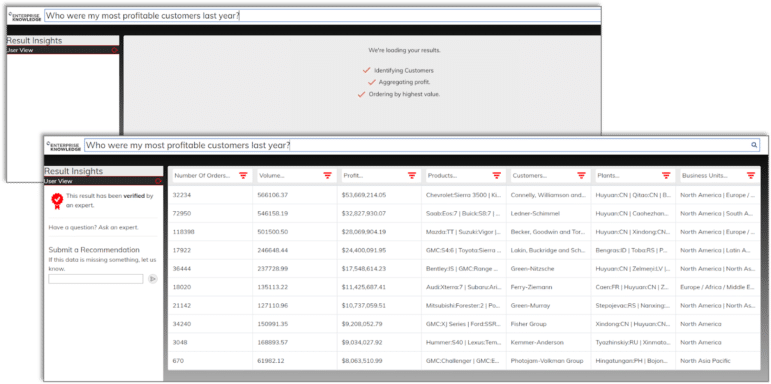
By allowing the company to collect, integrate, and identify user interest and intent, ontologies and knowledge graphs provided the foundation for Artificial Intelligence (AI), ultimately enabling the joint analysis of different entity paths, as well as the ability to describe their connectivity from various angles, and discover hidden facts and relationships through inferences in related content that would have otherwise gone unnoticed. For this particular supply chain and manufacturing company, by connecting internal data to analyze relationships and further mine disparate data sources, they now have a holistic view of products and services they can leverage to influence operational decisions. The interface through which they interact with the knowledge graph enables non-technical users to uncover the answers to a variety of critical business questions, such as:
- Which of your products or services are most profitable and perform better?
- What investments are successful, and when are they successful?
- How much of a given product did we deliver in a given timeframe?
- Who were my most profitable customers last year?
- How can we align products and services with the right experts, locations, delivery method, and timing?