Auto-tagging processes automate the manual labor of applying relevant keyword tags to data and content, enhancing accessibility and improving the organization of large datasets. Whether you’re trying to improve how quickly you find data or embarking on a content cleanup effort or you’re setting up a knowledge management system across an entire organization, these tools streamline the process and save you time. In this guide, we’ll explore the spectrum of auto-tagging options, from ready-made solutions to fully customized AI models, to help you find the perfect fit for your needs.
What is Auto-Tagging?
When managing large volumes of data, applying the right tags to clearly organize your information can feel like an endless task. Auto-tagging is an advanced process that automatically applies relevant terms/labels to your data. By identifying key information within a source and adding it as metadata, it makes organizing and finding data easy.
For organizations today, auto-tagging can streamline knowledge management systems, boost content/data personalization, and improve search engine optimization. Imagine automatically enabling customers to find exactly what they need from product manuals, categorizing thousands of marketing materials in just a few clicks, or categorizing research articles to help scientists locate relevant studies and data faster.
Auto-tagging isn’t just about efficiency—it can boost revenue by speeding up team workflows, cutting down search time, and enhancing customer experiences. It ensures that the right data reaches the right people, making it a smart choice for better business outcomes.
Do You Have an Existing Taxonomy or Metadata Model?
First things first, auto-tagging processes require an existing framework – either a taxonomy, or a metadata model, or both – to work from. Instead of starting from scratch and attempting to label your data from a blank slate, the auto-tagging process uses your taxonomy or metadata model to tap into your established framework and efficiently organize information and fine-tune keywords. If you don’t have a taxonomy or metadata model in place, don’t worry—it just means you’ll need to start by building one. To get started, you can review EK’s many resources on the topic: The 4 Steps to Designing an Effective Taxonomy, How Do I Implement A Taxonomy?, or Best Practices for Successful Metadata Governance. This is a crucial step to create a proper auto-tagging solution tailored to your unique data.
Auto-Tagging Approaches
When implementing auto-tagging, organizations can choose from a range of options that fall on a Spectrum of Customizability. On one end, you have Ready-Made Auto-Tagging Solutions, which are typically features housed within Taxonomy Management Systems (TMS) such as PoolParty, Semaphore, and TopBraid EDG. These plug-and-play tools offer quick setup, user-friendly features, and low-code/no-code interfaces, making them ideal for standard use cases. They also integrate easily if you are already using one of the tools listed above for taxonomy management, and require minimal upkeep. However, they come with limitations—customization options can be more restrictive, limited to the system’s current configuration capabilities, making them less suitable for unique business needs or specialized datasets like images or audio. Additionally, TMS often involve recurring licensing costs and lack transparency regarding model details, performance, and training methods. If these challenges don’t align with your project requirements, consider alternative approaches such as Custom-AI solutions, which are reviewed later in this blog.
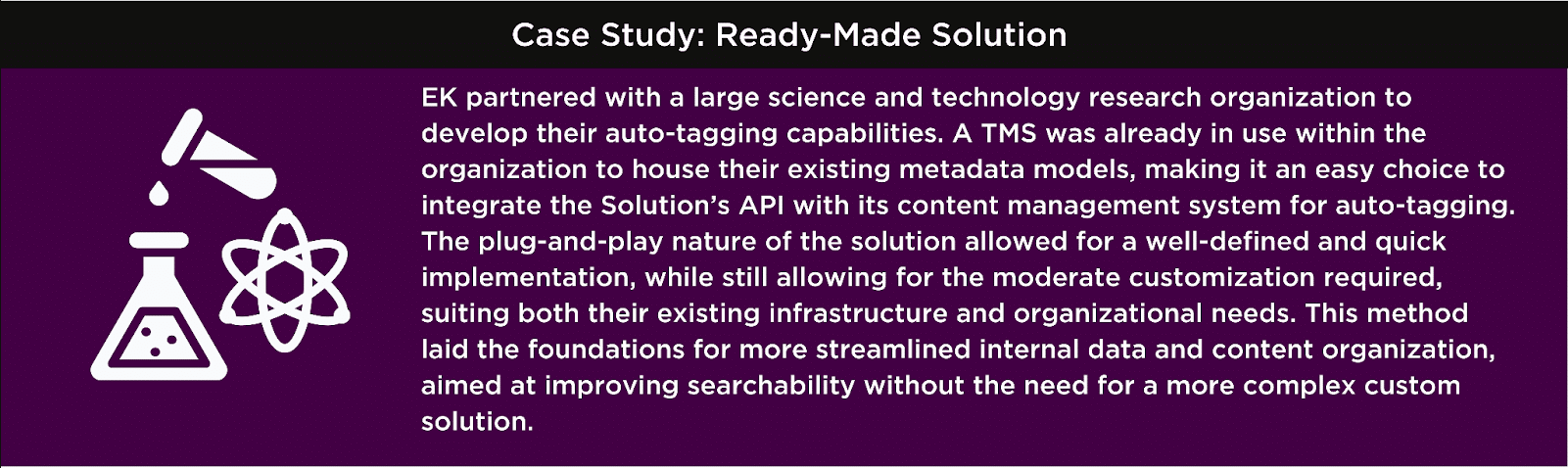
On the other end of the spectrum, there are Custom AI-Enabled Auto-Tagging solutions, where models are trained specifically on your business’s unique data for more specialized tagging needs. These solutions offer high levels of customization, greater accuracy for specific data types, and full transparency on how the model is built and performs. Once deployed, they avoid recurring costs outside of internal upkeep. The trade-off, however, is the requirement for significant technical expertise, high upfront costs, and a time-intensive data labeling and training process. These models also need regular updates and maintenance to ensure continued accuracy and performance.
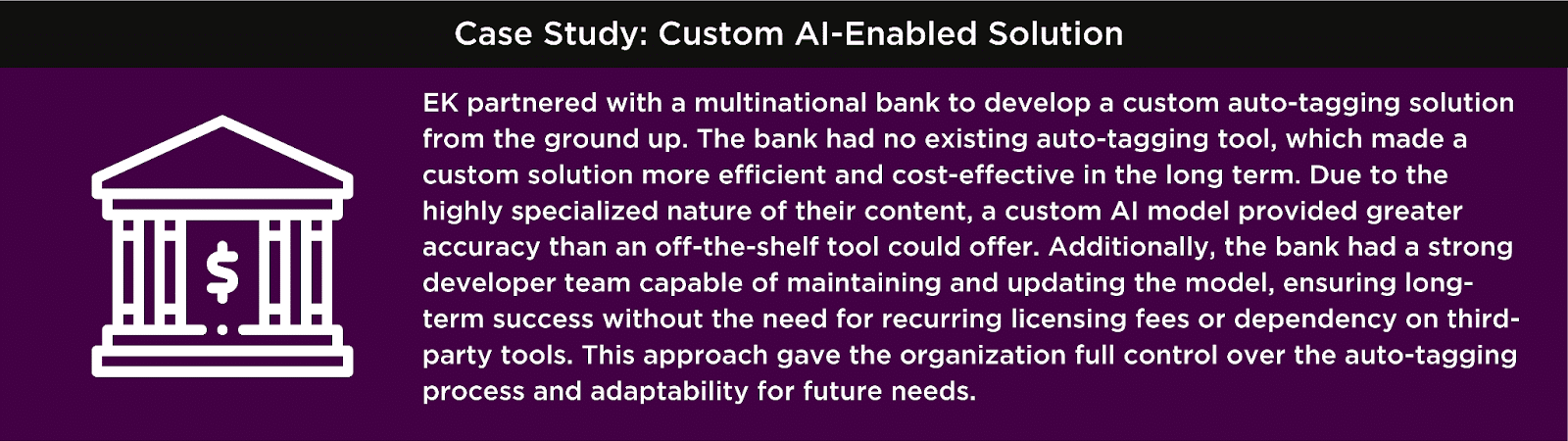
While each approach has its benefits, no solution is entirely hands-off—all solutions on the Spectrum of Customizability require human input to ensure accuracy, relevance, and usability. The right choice depends on your organization’s specific needs, data complexity, and level of control over the tagging process. The next few sections will dive into the specifics of Ready-Made vs Custom AI-Enabled Solutions and expand on the Spectrum of Customizability.
The Spectrum of Customizability
When it comes to auto-tagging, one size doesn’t fit all. Different organizations have unique data, needs, and resources, which means the right tagging solution can vary significantly. This is where the concept of customizability comes into play. Whether you need a simple, off-the-shelf solution or a highly tailored model, auto-tagging options exist on a spectrum of customizability, balancing ease of use with the ability to fine-tune for specific needs.
In this section, we’ll break down the levels of customizability, from pre-built solutions that are ready to go out of the box, to fully custom AI models that give complete control over tag generation and data processing. By understanding this spectrum, you can better assess which solution might work best for your use-case.
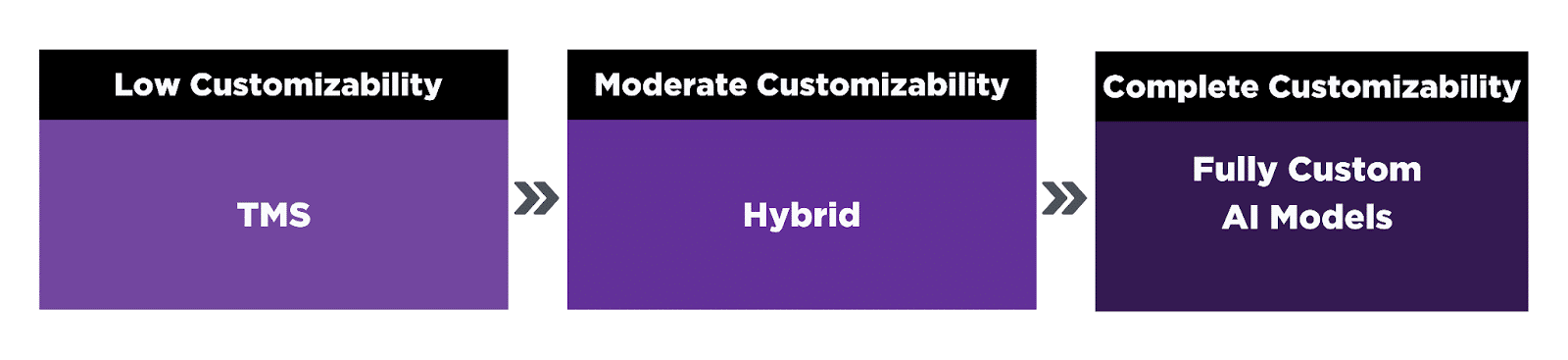
TMS | Hybrid | Custom AI | |
Description | These solutions are designed for rapid deployment, requiring minimal setup or configuration. However, you have little control over tag generation or model parameters. They’re best for simple use cases or highly standardized data. | These tools combine pre-built systems with the ability to integrate existing taxonomies, tweak configurations, or fine-tune pre-trained models using proprietary data. They balance ease of use with moderate customizability, enabling tailored tagging without full-scale development efforts. | These models are built from the ground up to meet highly specific tagging needs, starting with proprietary training data and leveraging traditional supervised learning techniques, offering complete control over the tagging logic. |
Level of Customizability | Low Customizability | Moderate Customizability | High Customizability |
Best For | Organizations that need a fast, simple tagging solution that works out of the box. | Organizations that need adaptable solutions for specific data contexts, with flexibility to adjust terms, tagging logic, and visibility on model types. | Organizations with highly specialized tagging needs that require complete control over the model, and are willing to invest in dedicated resources for maintenance. |
Conclusion
In summary, we’ve explored what auto-tagging is, how it can help your organization, and the auto-tagging Spectrum of Customizability, discussing benefits, challenges, and best use cases. If you’re unsure which method best fits your needs, or if you need help selecting a ready-made tool or developing an AI-enabled model, EK’s auto-tagging experts are here to assist. Don’t hesitate to reach out for personalized support!