In today’s data-driven world, managing taxonomies has become increasingly complex, requiring a balance between precision and usability. The Knowledge Intelligence (KI) framework – a strategic integration of human expertise, AI capabilities, and organizational knowledge assets – offers a transformative approach to taxonomy management. This blog explores how KI can revolutionize taxonomy management while maintaining strict compliance standards.
The Evolution of Taxonomy Management
Traditional taxonomy management has long relied on Subject Matter Experts (SME) manually curating terms, relationships, and hierarchies. While this time-consuming approach ensures accuracy, it struggles with scale. Modern organizations generate millions of documents across multiple languages and domains, and manual curation simply cannot keep pace with the large variety and velocity of organizational data while maintaining the necessary precision. Even with well-defined taxonomies, organizations must continuously analyze massive amounts of content to verify that their taxonomic structures accurately reflect and capture the concepts present in their rapidly growing data repositories.
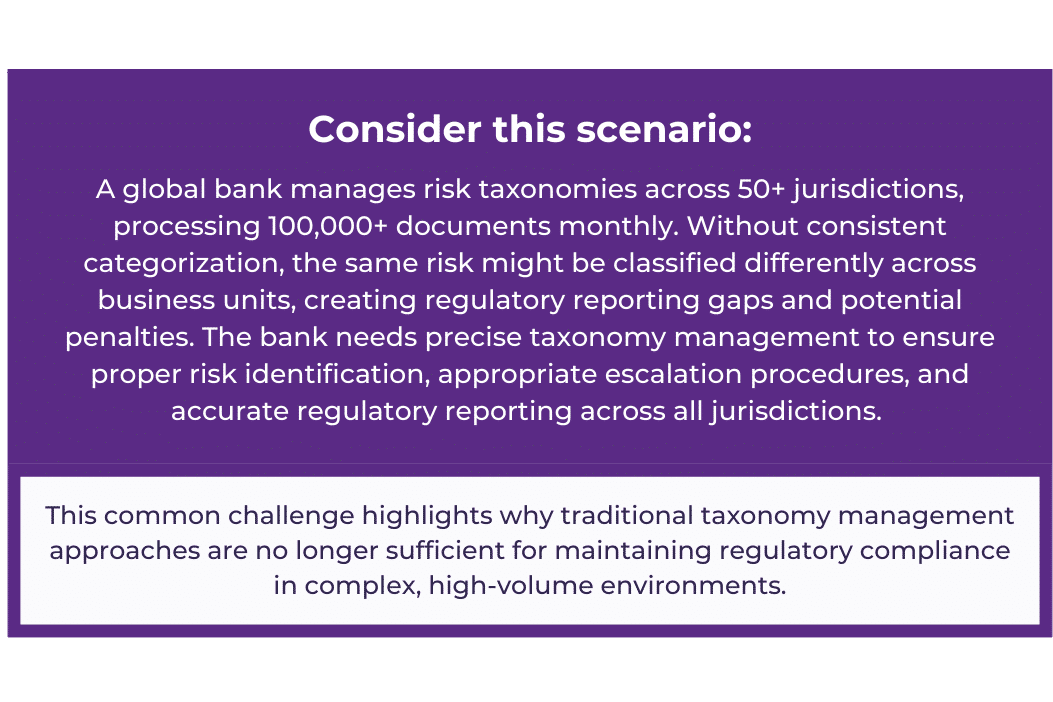
In the scenario above, traditional AI tools might help classify new documents, but an expert-guided recommender brings intelligence to the process.
KI-Driven Taxonomy Management
KI represents a fundamental shift from traditional AI systems, moving beyond data processing to true knowledge understanding and manipulation. As Zach Wahl explains in his blog, From Artificial Intelligence to Knowledge Intelligence, KI enhances AI’s capabilities by making systems contextually aware of an organization’s entire information ecosystem and creating dynamic knowledge systems that continuously evolve through intelligent automation and semantic understanding.
At its core, KI-driven taxonomy management works through a continuous cycle of enrichment, validation, and refinement. This approach integrates domain expertise at every stage of the process:
1. During enrichment, SMEs guide AI-powered discovery of new terms and relationships.
2. In validation, domain specialists ensure accuracy and compliance of all taxonomy modifications.
3. Through refinement, experts interpret usage patterns to continuously improve taxonomic structures.
By systematically injecting domain expertise into each stage, organizations transform static taxonomies into adaptive knowledge frameworks that continue to evolve with user needs while maintaining accuracy and compliance. This expert-guided approach ensures that AI augments rather than replaces human judgement in taxonomy development.
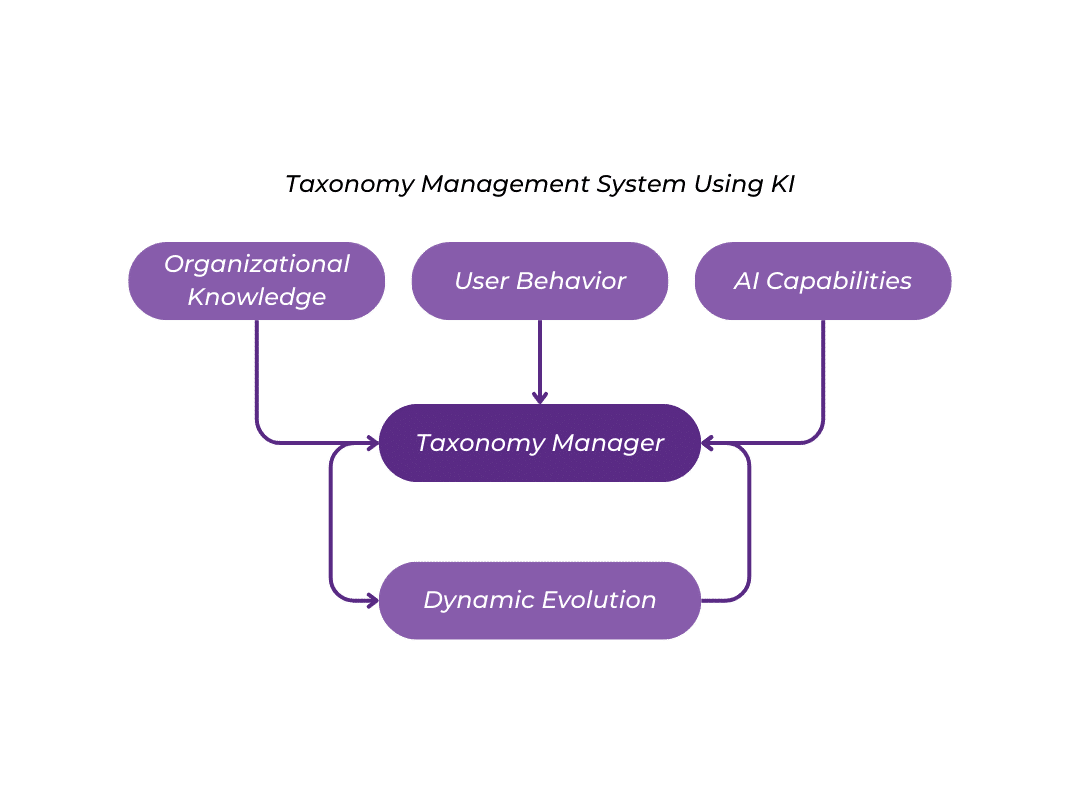
Enrichment: Augmenting Taxonomies with Domain Intelligence
When augmenting the taxonomy creation process with AI, SMEs begin by defining core concepts and relationships, which then serve as seeds for AI-assisted expansion. Using these expert-validated foundations, systems employ Natural Language Processing (NLP) and Generative AI to analyze organizational content and extract relevant phrases that relate to existing taxonomy terms.
Topic modeling, a set of algorithms that discover abstract themes within collections of documents, further enhances this enrichment process. Topic modeling techniques like BERTopic, which uses transformer-based language models to create coherent topic clusters, can identify concept hierarchies within organizational content. The experts evaluate these AI-generated suggestions based on their specialized knowledge, ensuring that automated discoveries align with industry standards and organizational needs. This human-AI collaboration creates taxonomies that are both technically sound and practically useful, balancing precision with accessibility across diverse user groups.
Validation: Maintaining Compliance Through Structured Governance
What sets the KI framework apart is its unique ability to maintain strict compliance while enabling taxonomy evolution. Every suggested change, whether generated through user behavior or content analysis, goes through a structured governance process that includes:
- Automated compliance checking against established rules;
- Human expert validation for critical decisions;
- Documentation of change justifications; and
- Version control with complete audit trails.
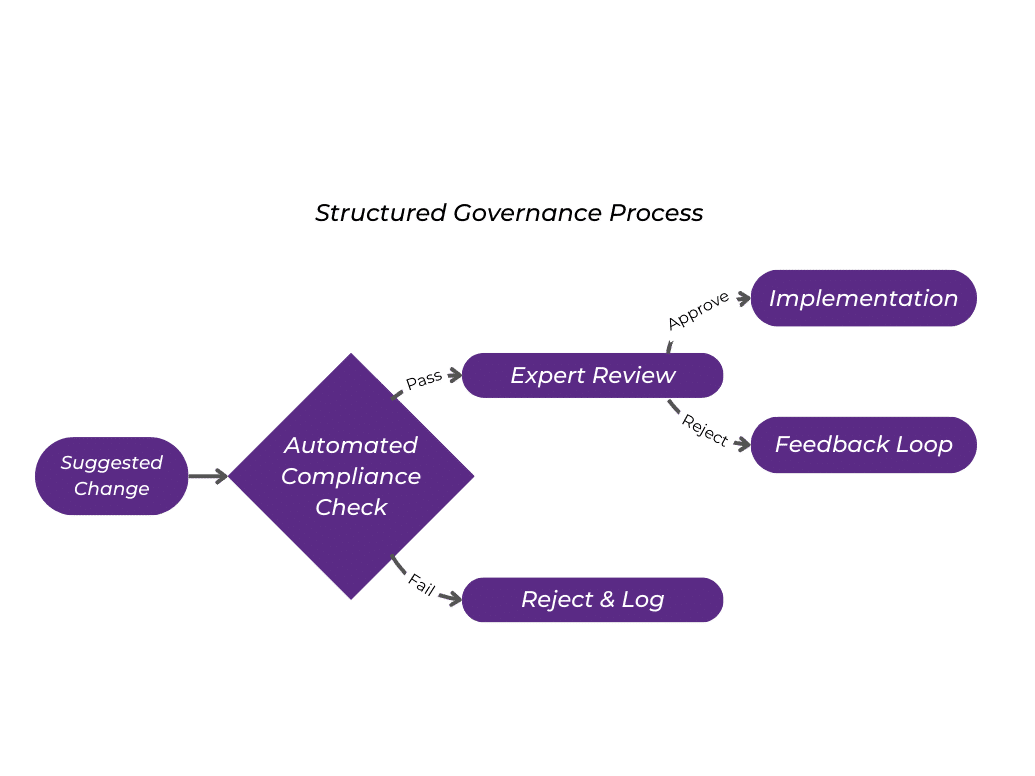
Organizations implementing KI-driven taxonomy management see transformative results including improving search success rates and decreasing the time required for taxonomy updates. More importantly, taxonomies become living knowledge frameworks that continuously adapt to organizational needs while maintaining compliance standards.
Refinement: Learning From Usage to Improve Taxonomies
By systematically analyzing how users interact with taxonomies in real-world scenarios, organizations gain invaluable insights into potential improvements. This intelligent system extends beyond simple keyword matching—it identifies emerging patterns, uncovers semantic relationships, and bridges gaps between formal terminology and practical usage. This data-driven refinement process:
- Analyzes search patterns to identify semantic relationships;
- Generates compliant alternative labels that match user behavior;
- Routes suggestions through appropriate governance workflows; and
- Maintains an audit trail of changes and justifications.
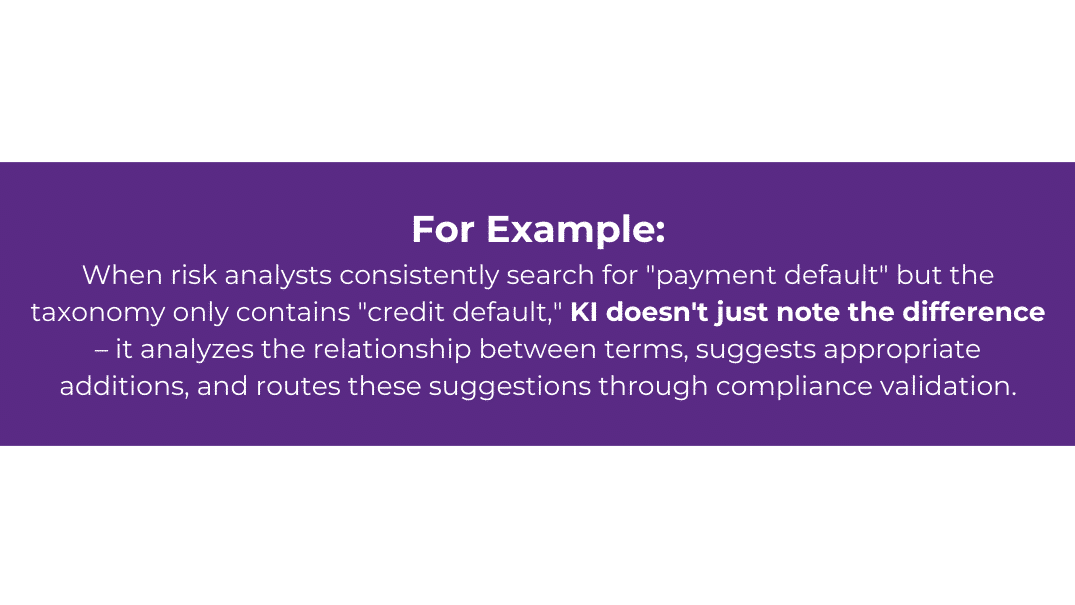
The refinement process analyzes the conceptual relationship between terms, evaluates usage contexts, and generates suggestions for terminological improvements. These suggestions—whether alternative labels, relationship modifications, or new term additions—are then routed through governance workflows where domain experts validate their accuracy and compliance alignment. Throughout this process, the system maintains a comprehensive audit trail documenting not only what changes were made but why they were necessary and who approved them.
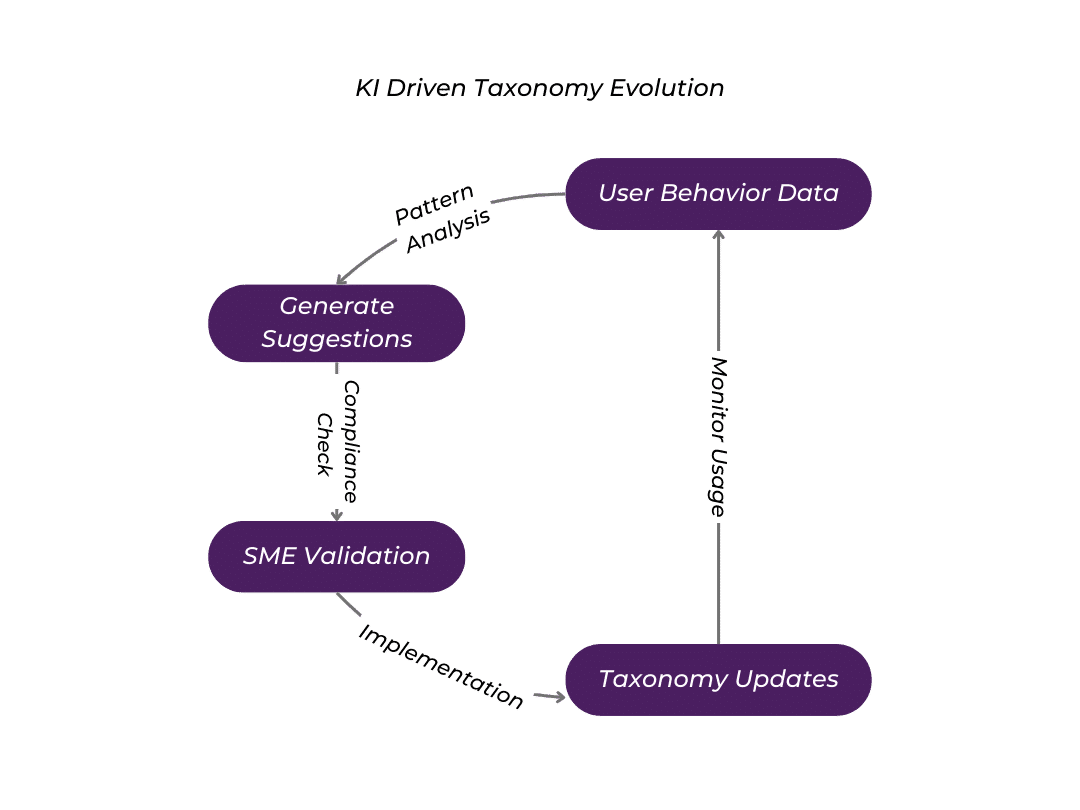
Case Study: KI in Action at a Global Investment Bank
To show the practical application of the continuous, knowledge-enhanced taxonomy management cycle, in the following section we describe a real-world implementation at a global investment bank.
Challenge
The bank needed to standardize risk descriptions across multiple business units, creating a consistent taxonomy that would support both regulatory compliance and effective risk management. With thousands of risk descriptions in various formats and terminology, manual standardization would have been time-consuming and inconsistent.
Solution
Phase 1: Taxonomy Enrichment
The team began by applying advanced NLP and topic modeling techniques to analyze existing risk descriptions. Risk descriptions were first standardized through careful text processing. Using the BERTopic framework and sentence transformers, the system generated vector embeddings of risk descriptions, allowing for semantic comparison rather than simple keyword matching. This AI-assisted analysis identified clusters of semantically similar risks, providing a foundation for standardization while preserving the important nuances of different risk types. Domain experts guided this process by defining the rules for risk extraction and validating the clustering approach, ensuring that the technical implementation remained aligned with risk management best practices.
Phase 2: Expert Validation
SMEs then reviewed the AI-generated standardized risks, validating the accuracy of clusters and relationships. The system’s transparency was critical so experts could see exactly how risks were being grouped. This human-in-the-loop approach ensured that:
- All source risk IDs were properly accounted for;
- Clusters maintained proper hierarchical relationships; and
- Risk categorizations aligned with regulatory requirements.
The validation process transformed the initial AI-generated taxonomy into a production-ready, standardized risk framework, approved by domain experts.
Phase 3: Continuous Refinement
Once implemented, the system began monitoring how users actually searched for and interacted with risk information. The bank recognized that users often do not know the exact standardized terminology when searching, so the solution developed a risk recommender that displayed semantically similar risks based on both text similarity and risk dimension alignment. This approach allowed users to effectively navigate the taxonomy despite being unfamiliar with standardized terms. By analyzing search patterns, the system continuously refined the taxonomy with alternative labels reflecting actual user terminology, and created a dynamic knowledge structure that evolved based on real usage.
This case study demonstrates the power of knowledge-enhanced taxonomy management, combining domain expertise with AI capabilities through a structured cycle of enrichment, validation, and refinement to create a living taxonomy that serves both regulatory and practical business needs.
Taxonomy Standards
For taxonomies to be truly effective and scalable in modern information environments, they must adhere to established semantic web standards and follow best practices developed by information science experts. Modern taxonomies need to support enterprise-wide knowledge initiatives, break down data silos, and enable integration with linked data and knowledge graphs. This is where standards like the Simple Knowledge Organization System (SKOS) become essential. By using universal standards like SKOS, organizations can:
- Enable interoperability between systems and across organizational boundaries
- Facilitate data migration between different taxonomy management tools
- Connect taxonomies to ontologies and knowledge graphs
- Ensure long-term sustainability as technology platforms evolve
Beyond SKOS, taxonomy professionals should be familiar with related semantic web standards such as RDF and SPARQL, especially as organizations move toward more advanced semantic technologies like ontologies and enterprise knowledge graphs. Well-designed taxonomies following these standards become the foundation upon which more advanced Knowledge Intelligence capabilities can be built. By adhering to established standards, organizations ensure their taxonomies remain both technically sound and semantically precise, capable of scaling effectively as business requirements evolve.
The Future of Taxonomy Management
The future of taxonomy management lies not just in automation, but in intelligent collaboration between human expertise and AI capabilities. KI provides the framework for this collaboration, ensuring that taxonomies remain both precise and practical.
For organizations considering this approach, the key is to start with a clear understanding of their taxonomic needs and challenges, and to ensure their taxonomy efforts are built on solid foundations of semantic web standards like SKOS. These standards are essential for taxonomies to effectively scale, support interoperability, and maintain long-term value across evolving technology landscapes. Success comes not from replacement of existing processes, but from thoughtful integration of KI capabilities into established workflows that respect these standards and best practices.
Ready to explore how KI can transform your taxonomy management? Contact our team of experts to learn more about implementing these capabilities in your organization.