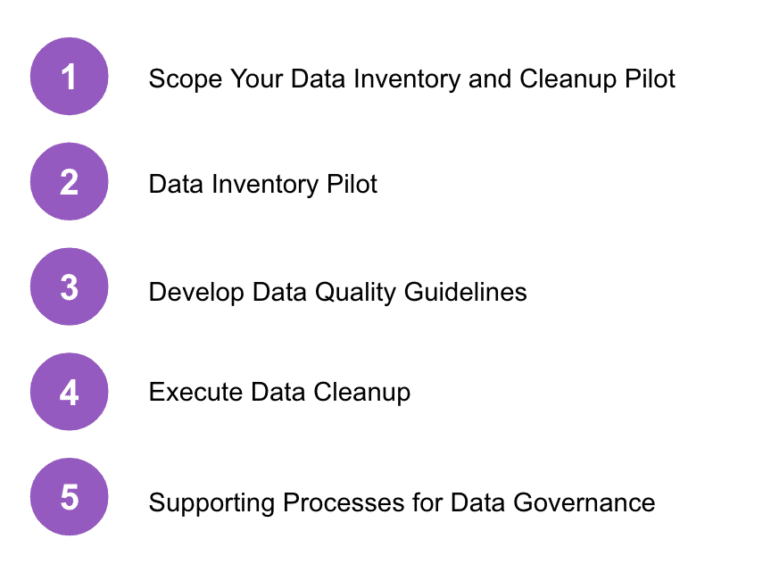
At EK, many of the challenges we hear about revolve around data – no matter the company’s size or industry. Without clean, centralized data, staff may begin to lose trust and confidence in the information they are working with. They may have trouble conducting effective data analysis and extracting data insights, and the organization as a whole remains ill-prepared for more advanced applications of data, such as knowledge graphs, artificial intelligence (AI), and machine learning (ML). Each of these business challenges is due to ineffective data management and governance.
Organizations with siloed and inconsistent data need an enterprise data architecture and governance model – but where do you start? How can you make small, iterative steps to see impact quickly, validate assumptions, and ensure successful rollout across the enterprise? This blog describes an approach to data cleanup and management, ultimately leading to enterprise-wide data integrity and standards.
Step 1: Scope Your Data Inventory and Cleanup Pilot
A data inventory allows an organization to gain a better understanding of the current state of its data, thus supporting a future data cleanup based on the findings and insights. For many organizations this is an expensive and time consuming exercise. The key success factor here is to start small. Factors to consider when prioritizing the data for your pilot include:
- Risk: Is the data regulated or subject to privacy considerations?
- Business value: Is this data a key indicator of business goals (e.g., revenue, user engagement)?
- Visibility: To what degree will the pilot data attract attention throughout the organization?
- User buy-in: How eager and enthusiastic would users be for a pilot leveraging this data?
Accessibility of data: How easily accessible is the data? Are there security considerations?
Step 2: Data Inventory Pilot
A data inventory will permit your organization to gain a clear understanding of how users access, use, and collaborate with the chosen system(s), thus beginning to identify relevant data quality and data management issues, concerns, and considerations.
With the inventory, your organization will have an overview of relevant data sources and data elements and can then use this inventory to draw insights, such as initial areas for enrichment and the early identification of data management challenges.
Inventory of sample data mapped to common data challenges.
Step 3: Develop Data Quality Guidelines
The team should seek to address data quality challenges surfaced in the data inventory with clear, actionable cleanup processes and data quality guidelines that establish standards for the areas of inconsistency and risk within your data inventories. While these guidelines will initially focus on the prioritized systems, your organization can reap even greater value by scaling these guidelines and evolving them across the organization beyond this pilot activity.
Sample data quality issues and their corresponding guidelines and cleanup actions.
Step 4: Execute Data Cleanup
It is essential to prioritize data cleanup and enrichment areas to focus on, with the end goal of cleaning up, archiving, deleting, and/or migrating data assets based on your standardized and replicable data cleanup guidelines.
A cleanup can be completed through a mix of manual and automated methods. Auto-tagging is one method that can help automate the process, through automatically classifying assets based on predetermined sets of terms that identify the assets as candidates for further action or cleanup. Overall, executing the data cleanup will result in more standardized, reliable, and accessible data at the organization.
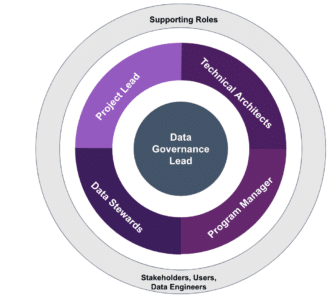
Step 5: Supporting Processes for Data Governance
It’s important to develop a data governance framework to better maintain, control, and update your data, cementing the value gained through your data cleanup. These governance rules and processes should help to eliminate duplicative data and allow staff to easily maintain data assets, helping them to better trust the information they are finding in downstream applications.
The framework should seek to address the user personas responsible for aspects of data governance, such as data owners, data stewards, data analysts, and project leads, and the recommended actions users should take to address common pitfalls with data quality and any identified enrichment challenges.
Conclusion
Through executing a data cleanup pilot end-to-end, you will see the value in making impactful improvements to the quality and reputability of your data, and can leverage lessons learned to execute additional data cleanup across the enterprise. EK can help you operationalize your data strategy initiatives through designing and executing a set of scalable data cleanup pilots. Ready to see the impact of higher quality data? Contact us.