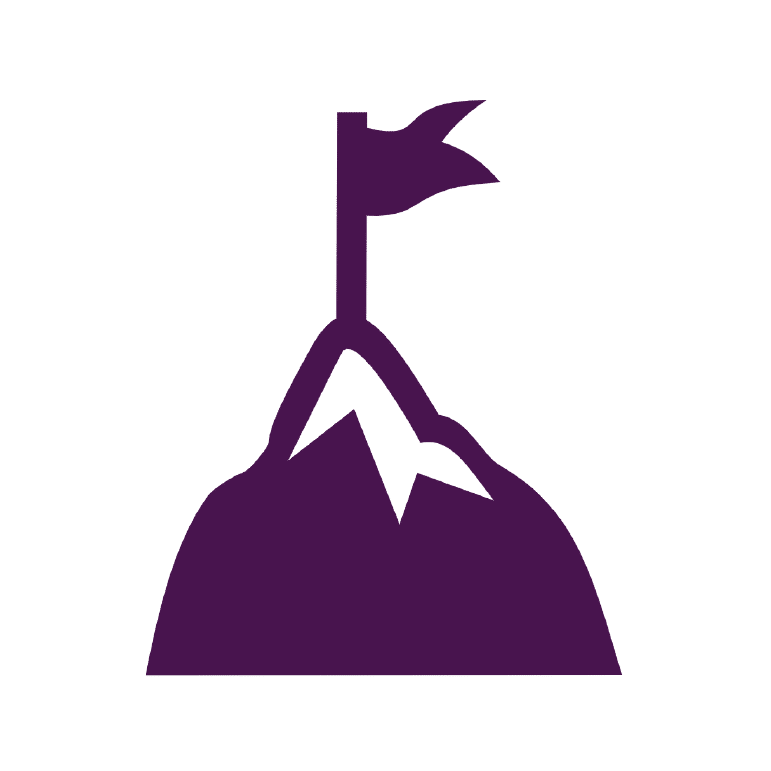
The Challenge
A bioscience technology provider – and a leader in scientific research and solutions – identified a pivotal challenge within their digital ecosystem, particularly on their public facing e-commerce website. While the platform held an extensive reservoir of both product information and associated educational content, the content and data existed disjointedly (spread across more than five systems). As a result, their search interface failed to offer users a holistic and enriching experience. A few primary issues at hand were:
- The search capability was largely driven by keywords, limiting its potential to be actionable.
- The platform’s search functionality didn’t seamlessly integrate all available resources, leading to underutilized assets and a compromised user experience.
- The painstaking manual process of collating content posed internal challenges in governance and hindered scalability.
- In the absence of a cohesive content classification system, there was a disjunction between product information and corresponding educational content.
- Inconsistencies plagued the lifecycle management of marketing content.
- The array of platforms, managed by different product teams, exposed alignment challenges and prevented a unified user experience.
From a business perspective, the challenges were even more dire. The company faced potential revenue losses as users couldn’t gain enough insight to make buying decisions. The user experience became frustrating due to irrelevant content and inefficient searches, limiting employees with manual processes and impeding data-driven decision-making regarding the value of the site’s content; this caused both employees and customers to resort to doing Google searches that routed them back to the site to find what they needed.
The company engaged EK to help bridge the gap between product data and marketing and educational content to ultimately improve the search experience on their platform.
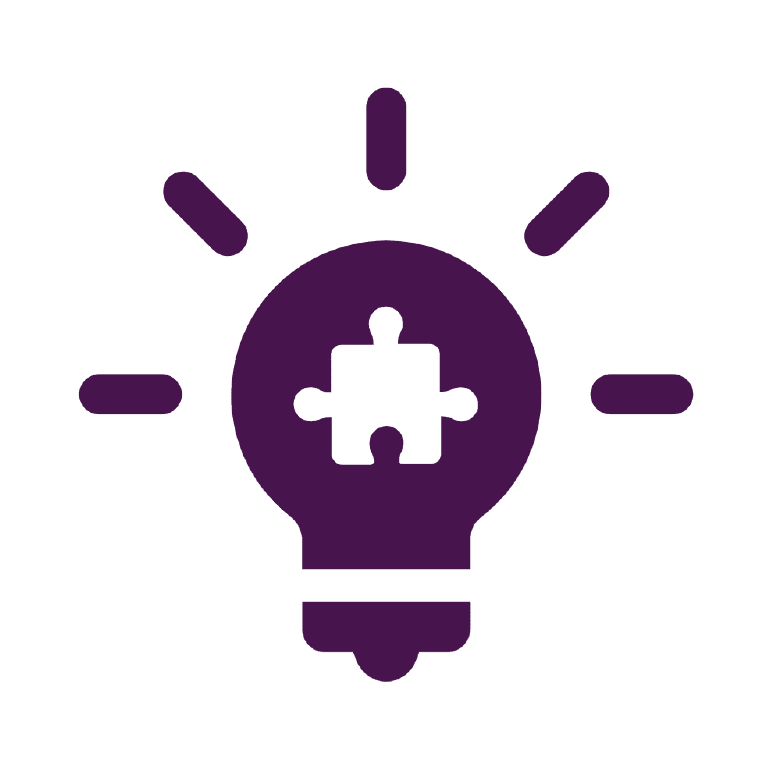
The Solution
Assessing Current Content and Asset Capabilities at Scale
EK commenced its engagement by comprehensively assessing the company’s current content and asset capabilities. This deep dive included a data mapping and augmented corpus analysis effort into the content and technologies that power their website, such as Adobe AEM (marketing content), a Learning Management System (LMS) with product-related educational content, a Product Information Management (PIM) solution with over 70,000 products, and Salesforce for storing customer data. This provided a clear picture of the existing content and data landscape.
A Semantic Data Model
With a deeper understanding of the content’s diversity and the need for efficient classification, EK defined and implemented a robust taxonomy and ontology system. This provided a structured way to classify and relate content, making it more discoverable and actionable for users. To tangibly demonstrate the potential of knowledge graphs, EK implemented a POC. This POC aimed to bridge the silos between the different systems, allowing for a more unified and cohesive content experience that connected product and marketing information.
Integrated Data Resources and Knowledge Graph Embeddings
EK utilized an integrated data set to counter data fragmentation across different platforms. A more cohesive content resource was built by combining Adobe AEM and LMS data with manually curated data and extracted information from the rendered website. However, the critical leap came when the entire knowledge graph, which encapsulated this unified data set, was loaded into memory. This in-memory knowledge graph paved the way for real-time processing and analysis, which is essential for generating meaningful embeddings.
Similarity Index and Link Classifier: Two-Fold Search Enhancement
- Similarity Index: EK’s Enterprise AI and Search experts worked together to convert the in-memory knowledge graph into vector embeddings. These embeddings, teeming with intricate data relationships, were harnessed to power a similarity index; this index stands as a testament to AI’s potential, offering content recommendations rooted in contextual relevance and similarity metrics.
- Link Classifier: Building upon the embeddings, EK introduced a machine learning (ML) classifier. This tool was meticulously trained to discern patterns and relationships within the embeddings, establishing connections between products and content. Consequently, the system was endowed with the capability to recommend content corresponding to a user’s engagement with a product or related content. This transformed the user journey, enriching it with timely and pertinent content suggestions.
ML-Infused User Experience Enhancement
Venturing beyond conventional methodologies, EK incorporated ML, knowledge graphs, taxonomy, and ontology to redefine the user experience. This allowed users to navigate and discover important content through an ML-powered content discovery system, yielding suggestions that resonated with their needs and browsing history.
Unified Platform Management via Predictive Insights
Addressing the multifaceted challenge of various teams steering different platforms, EK integrated the machine learning classifier with predictive insights. This fusion empowered teams with the foresight to gauge user preferences, allowing them to align platform features and fostering a cohesive and forward-looking digital landscape.
Search Dashboard Displaying ML-based Results
Concluding their engagement, EK presented with a search dashboard. This dashboard, designed to exhibit two distinct types of results – similarity index and link classifier – served as a window for the organization to witness and evaluate the dual functionalities. The underlying intent was to grant their e-commerce website backend avenues to elevate their search capabilities, giving them a comparative view of multiple ML-based systems.
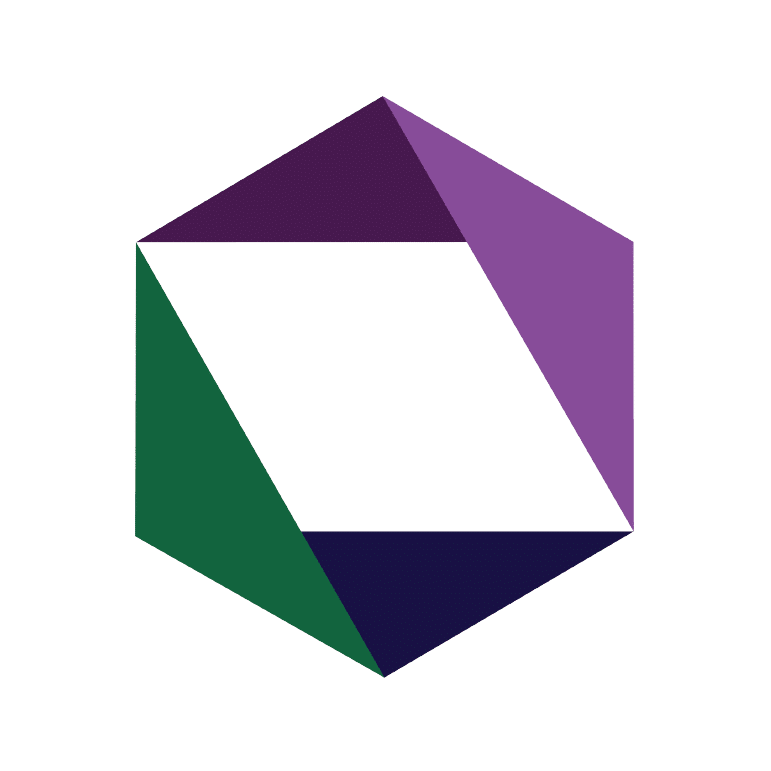
The EK Difference
EK’s hallmark is rooted in the proficiency of advanced AI and knowledge graph technologies, as well as our profound commitment to client relationships. Working closely with the company’s content and data teams, EK displayed a robust understanding of the technological necessities and the organizational dynamics at play. Even when the level of effort and need from the solution extended beyond the initial scope of work, EK’s flexible approach allowed for open dialogue and iterative development and value demonstration. This ensured that the project’s progression aligned closely with the evolving needs of our client.
Recognizing the intricacy of the project and the importance of a well-documented process, EK meticulously enhanced the documentation of both the delivery process and development. This created transparency and ensured that all the resources needed to carry forward, modify, or scale the implemented solution are in place for the future.
Moreover, given the complexity and nuances involved in such large-scale implementations, EK provided a repeatable framework to validate AI results with stakeholders and maintain integrity and explainability of solutions with human-in-the-loop development throughout the engagement. This was achieved through iterative sessions, ensuring the final system met technical benchmarks and resonated with the company’s organizational context and language.
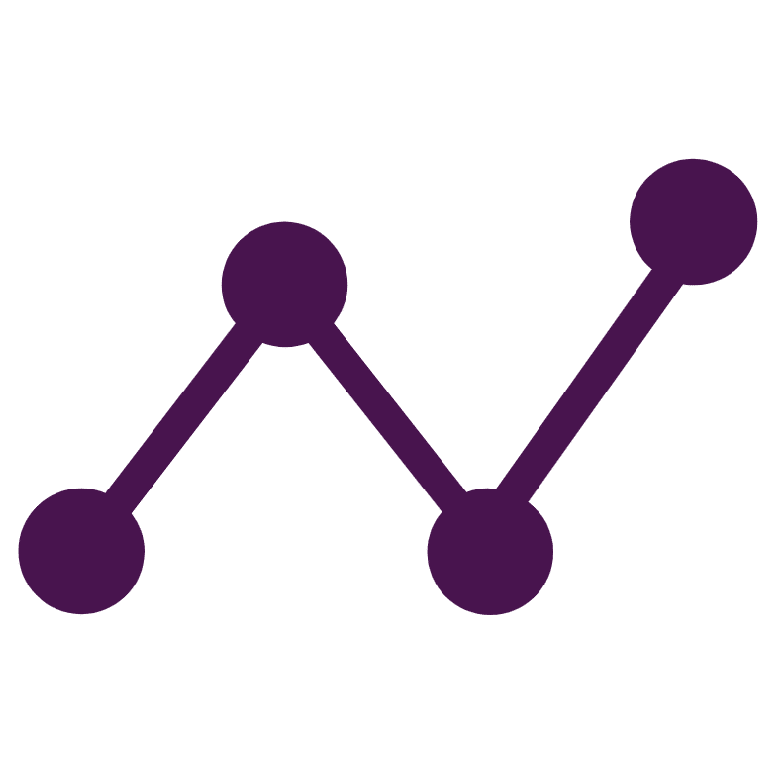
The Results
The engagement equipped the organization with a state-of-the-art, context-based recommendation system specifically tailored for their vast and diverse digital ecosystem. This solution drastically improved content discoverability, relevance, and alignment, fundamentally enhancing the user experience on their product website.
The exploratory nature of the project further unveiled opportunities for additional enhancements, particularly in refining the data, optimizing the system and exposing areas where the firm had gaps in content creation or educational materials as it relates to products. Other notable results include:
- Automated framework to standardized metadata across systems for over 70,000 product categories;
- A Proof of Concept (POC) that bridged content silos across 4+ different systems, demonstrating the potential of knowledge graphs;
- A machine-learning classifier that expedited content aggregation and metadata application process through automation; and
- Increased user retention and better product discovery, leading to 6 figures in closed revenue.