Content is a critical organizational asset. Whether product documentation, sales and marketing materials, industry rules and regulations, employee policies, or learning materials, content forms the backbone of operations, decision-making, and end-user experiences. Organizations are challenged to generate content and structure and manage it effectively for efficiency, discoverability, and compliance. The semantic layer addresses this challenge by adding context to content, capturing its relationships, and integrating it with organizational data into an integrated view.
Understanding the Semantic Layer
Before we explore the role of content in the semantic layer, a quick refresher on what a semantic layer is. In a recent post by EK’s Lulit Tesfaye, Partner and the VP for Knowledge & Data Services and Engineering, she proposes this definition:
A semantic layer is a standardized framework that organizes and abstracts organizational data (structured, unstructured, semi-structured) and serves as a data connector for data and knowledge. Larger than a data fabric, which is more focused on structured data, a semantic layer connects all organizational knowledge assets via a well-defined and standardized semantic framework, including content items, data, files, and media. It allows organizations to represent organizational knowledge and domain meaning to systems and applications, defining the relationship between content and data.
Beyond the data fabric, a semantic layer that incorporates content makes it possible to organize and enrich content with semantic meaning, empowering consuming systems and end users with advanced content management, discovery, and analytical capabilities.
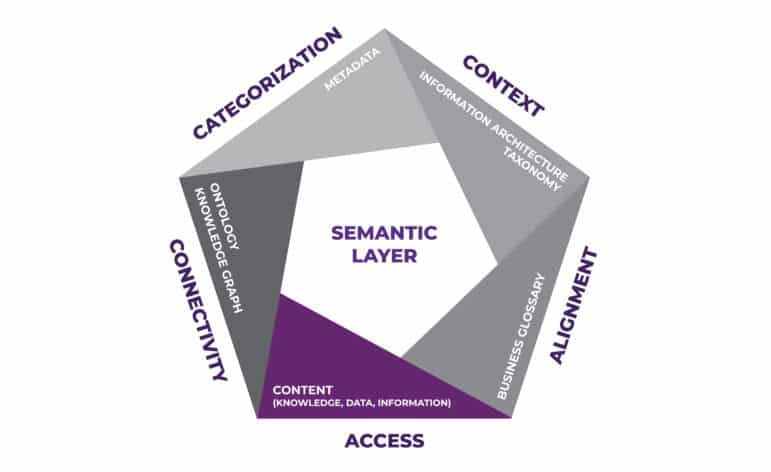
Enriching Content in the Semantic Layer
The semantic layer provides contextual understanding to content by capturing the meaning, relationships, and domain-specific knowledge embedded within it by incorporating data about the content. Through techniques such as semantic tagging and entity extraction, the content is enriched with metadata that describes its context, topics, and entities. This contextual understanding enables users to interpret and analyze content and other integrated data sources in a more meaningful way, leading to deeper insights and better decision-making.
When content is broken down into a dynamic content model, it becomes possible to realize the benefits of the semantic layer at a granular level. Dynamic content lets you use your content as data and realize the full value of your business assets by making those structured content elements available to various consuming systems and experiences. When those structured content elements are individually enriched with metadata like tags, categories, and keywords, they become building blocks that can be targeted and assembled at the point of need, creating contextually relevant content experiences. The semantic layer allows you to find the content that has an answer and directs you to the answer itself. Combining dynamic content and the semantic layer creates opportunities for improved content operations processes through the content lifecycle. Examples of content that can be managed in this structured manner include rules and regulations, employee handbooks, technical documentation, sales enablement and product marketing copy, and learning content.
Content Operations and the Semantic Layer
Content integrated into a semantic layer supports the capabilities of content operations systems in several ways. The content can be better structured and organized in a content or digital asset repository for more efficient and relevant access. Recommendation engines can use the data to deliver content that is aligned with user interests and needs. The Entity recognition and topic modeling facilitates content analysis to inform strategy and decision-making. When fed into consuming systems, the combination of the content and the semantic layer facilitates effective data management, knowledge discovery, and decision-making processes within an organization.
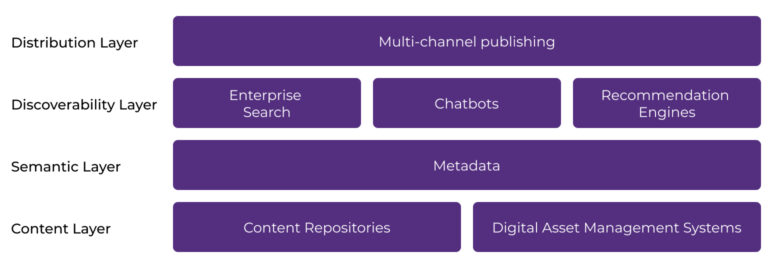
When content is incorporated into a semantic framework, organizations have a powerful tool for unlocking the full potential of their content investment as it is integrated with the full range of organizational data and knowledge.
Use Cases for Content in the Semantic Layer
The need for actionable insights and efficient information retrieval can be supported by the integration of a content graph within a semantic layer, consolidating disparate content sources into a cohesive knowledge repository. This integration process incorporates standardized metadata and establishes mappings of the relationships and dependencies between different concepts and entities. It facilitates the automation of content generation processes, leveraging advanced algorithms and structured data to produce tailored content at scale.
Let’s look at how this capability can enable applications in knowledge discovery, automated content generation, and content recommendations.
Knowledge Discovery
Represented through ontologies, taxonomies, and knowledge graphs, the content within the semantic layer embodies organizational knowledge and domain expertise. This rich knowledge representation facilitates exploration and discovery, allowing users to uncover valuable insights and patterns hidden within interconnected content.
When that content is modeled and structured in the source systems, it can be enriched with metadata at the component level. The structure of content in a content repository plays a crucial role in facilitating data organization and abstraction within the semantic layer regardless of its original format or source. By incorporating the content metadata into a common semantic framework with other types of data, the semantic layer enables integration and interoperability between different data types, allowing users to access and leverage all relevant information from a single source. That source is then optimized for the kind of system that consumes it, whether a web content management system, a learning management system, a marketing campaign management system, or another use case.
In a recent project, EK worked with a federal space research institute to develop an enterprise knowledge graph to connect the dots between people, projects, engineering components, and engineering topics. Leveraging and enriching an existing taxonomy and ontology at the organization, EK automatically extracted key entities from a repository of unstructured documents, adding structured metadata to these text files. That knowledge graph was then incorporated into a semantic search platform to enable faceted search and navigation across individuals, projects, and unstructured text documents, significantly reducing time spent finding information.
Automated Content Generation
Managing content within a semantic layer can also facilitate content generation for highly structured content types such as those used in industries such as pharma (e.g., rules, regulations, definitions, reports). By organizing content elements and their relationships in a structured format, the semantic layer provides the foundation for understanding the context and meaning of data. This contextual understanding enables intelligent algorithms to analyze existing content, identify patterns, and generate new content autonomously.
Using Natural Language Processing (NLP) techniques, algorithms can extract insights from structured content and generate human-readable text based on predefined templates or rules, such as articles, reports, or summaries. By leveraging machine learning models trained on vast amounts of data, these algorithms can adapt and improve over time, producing increasingly accurate and relevant content.
Additionally, the semantic layer facilitates the reuse of existing content components in a structured content environment, such as paragraphs, sections, or entire documents, to create new content. Automated systems can dynamically assemble tailored content pieces by identifying and retrieving relevant content based on semantic relationships and metadata.
The combination of structured content management in a semantic layer and advanced algorithms for automated content generation opens up possibilities for streamlining content creation processes, reducing manual effort, and delivering personalized and timely content at scale.
EK recently worked with a pharmaceutical company to automate regulatory report generation. We created an ontological data model and knowledge graph to map experiment management and results data across the product development lifecycle. By extracting and connecting information in the semantic layer, analysts can quickly generate reports needed for regulatory filings and use templatized content models to embed those reports seamlessly.
Content Recommendations
The semantic layer enriches content with additional metadata and annotations to enhance its discoverability, relevance, and usability. By analyzing content for relevant keywords, topics, and sentiment, the semantic layer enables targeted content recommendations and tailored user experiences. Leveraging ontologies and knowledge graphs, content can be organized within the semantic layer, making it easier to surface relevant content to users based on their interests and browsing history. Through continuous analysis of user interactions and feedback, content recommenders can adapt and refine their recommendations in real time, ensuring relevance and timeliness.
With content integrated into the semantic layer, organizations can implement next-generation content recommenders, offering hyper-personalized experiences by leveraging enriched metadata and advanced algorithms to support targeted content delivery and recommend similar content. Personalized content recommendations increase user satisfaction and drive higher engagement metrics such as click-through rates, time spent on site, and conversion rates.
Semantic layers can identify related content items by analyzing the similarity between pieces of content. This similarity analysis enables the creation of features such as “You May Also Like” recommendations or relevant course materials based on user identity and behavior, enhancing engagement and content discovery.
EK recently worked with an organization that provides online healthcare compliance training solutions to build a cloud-based recommendation engine microservice supported by a semantic data layer that unifies, relates, and organizes the source data (course titles, descriptions, tags, and more). The engine generates recommendations for compliance terms for tagging content to appear in recommended courses for customers to ensure that they comply with regulations critical to their role, setting, and jurisdiction.
Summary
Structuring and organizing content within the semantic layer empowers organizations to extract valuable insights, standardize metadata, identify relationships, and enhance searchability. This facilitates the seamless integration of diverse content sources into applications such as recommendation engines, dashboards, and end-user-facing content. This integration empowers users to access and leverage all relevant information from a single source, driving better decision-making and knowledge discovery.