Artificial Intelligence (AI) enables organizations to leverage and manage their content in exciting new ways, from chatbots and content summarization to auto-tagging and personalization. Most organizations have a copious amount of content and are looking to use AI to improve their operations and efficiency while enabling end users to find relevant information quickly and intuitively.
With the rise of ChatGPT and other generative AI tools in the last year, there’s a common misconception that you can “do” AI on any content with no preparation. If you want accurate and useful results and insights, however, it requires some upfront work. Understanding how AI interacts with your content and how your content strategy supports AI readiness will set you up for an effective AI implementation.
How AI Interacts with Content
While AI can help in many phases of the content lifecycle, from planning and authoring to discovery, AI usually interacts with existing content in two key ways:
1) Comprehension: AI must parse existing content to “understand” an organization’s vernacular or common language. This helps the AI model create statistical models, cluster content and concepts, and create a baseline for addressing future inputs. | |
2) Search: AI often needs to quickly identify snippets of content related to text, chunking longer content into smaller components and searching these components for relevant material. These smaller snippets are often used to gain an understanding of new or updated material. |
When AI examines existing content, it is trying to understand what it is about and how it relates to other concepts within the knowledge domain, and there are steps we can take to help. While this blog is mostly considering how large language models (LLMs) and retrieval augmented generation (RAG) AI interact with content, the steps listed below will prepare content for a variety of other types of AI for both insight and action.
Developing a Content Strategy
The best way to prepare content for AI is to develop a content strategy that addresses the relationships, the structure, the clean up, and the componentization of the content. One key preliminary activity will be to audit your content with the specific lens of AI-readiness, and to assess your organization’s content against the steps listed below.
Model the Knowledge Domain
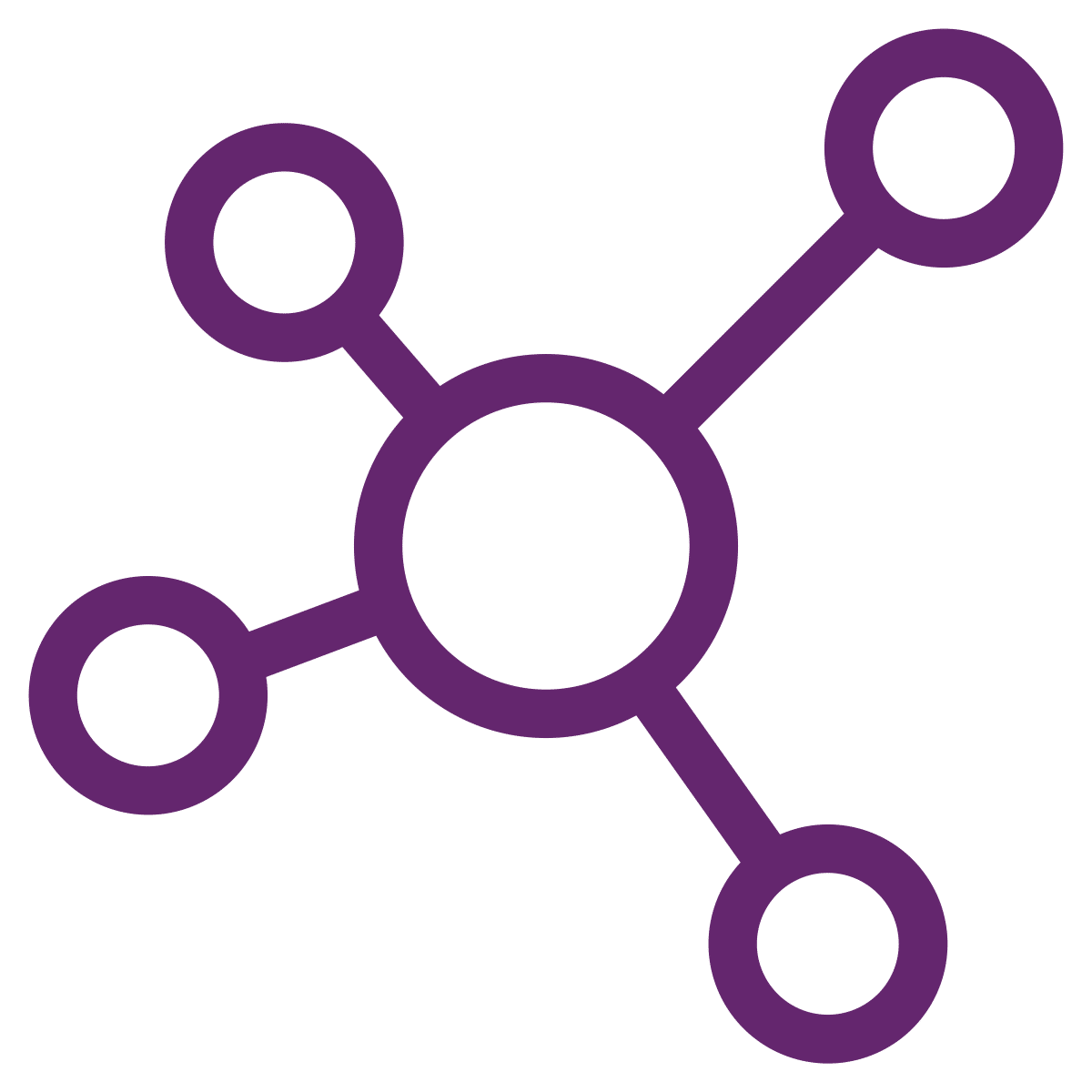
In most situations, AI creates internal models to group and cluster information to help the AI respond efficiently to new inputs. AI does a decent job of inferring the relationships between information, but organizations can significantly assist this process by defining an ontology. Ontologies enable organizations to define and relate organizational information, codifying how people, tools, content, topics, and other concepts are related. These models improve findability, support advanced search use cases, and form semantic layers that facilitate the integration of data from multiple sources into consumable formats and user-intuitive structures.
Once created, an ontology can be used with content to:
Modeling an organization’s knowledge domain with an ontology improves AI’s ability to utilize content more effectively and produce more accurate results.
Cleanup and Deduplicate the Content
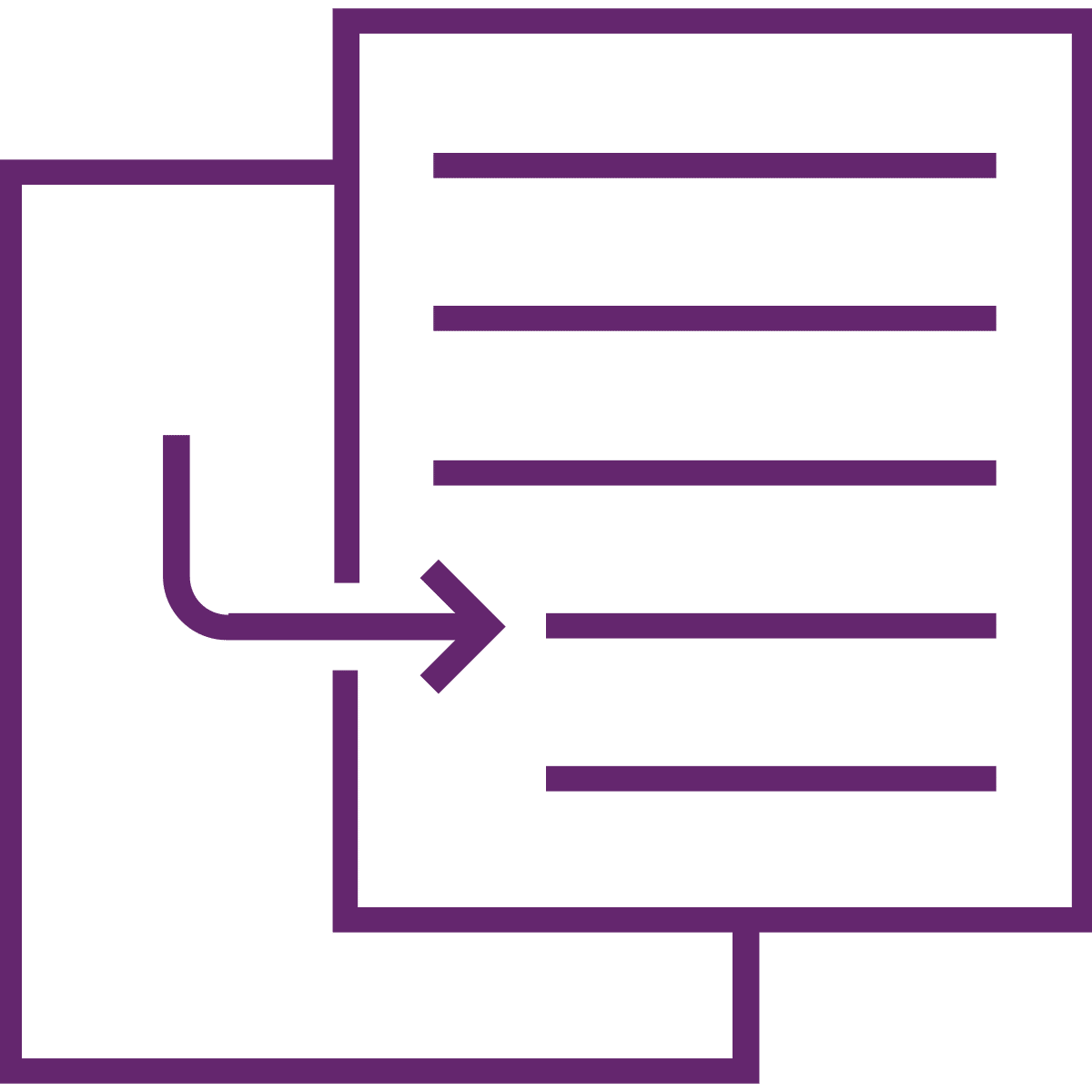
Today’s organizations have too much content and duplicated information. Content is often split between multiple systems due to limitations with legacy tools, user permissions, or the need to support new features and displays. While auditing all of an organization’s content may seem daunting, there are steps an organization can take to streamline the process. Organizations should focus on their NERDy content, identifying the new, essential, reliable, and dynamic content users need to perform their jobs. As part of this focus, organizations reduce content ROT (Redundant, Outdated, Trivial), improving user trust and experience with organizational information.
As part of the cleaning effort, an organization may want to create a centralized authoring platform to maintain content in one place rather than siloed locations. This allows content to be managed in one place, reducing the effort to update content and enabling content reuse. Reusing content helps deduplicate content, removing the need to replicate and update content in multiple places. A content audit, analysis, and clean-up will organize content in an intuitive way for AI and reduce bias from repeated or incorrect information.
Add Structure and Standardization
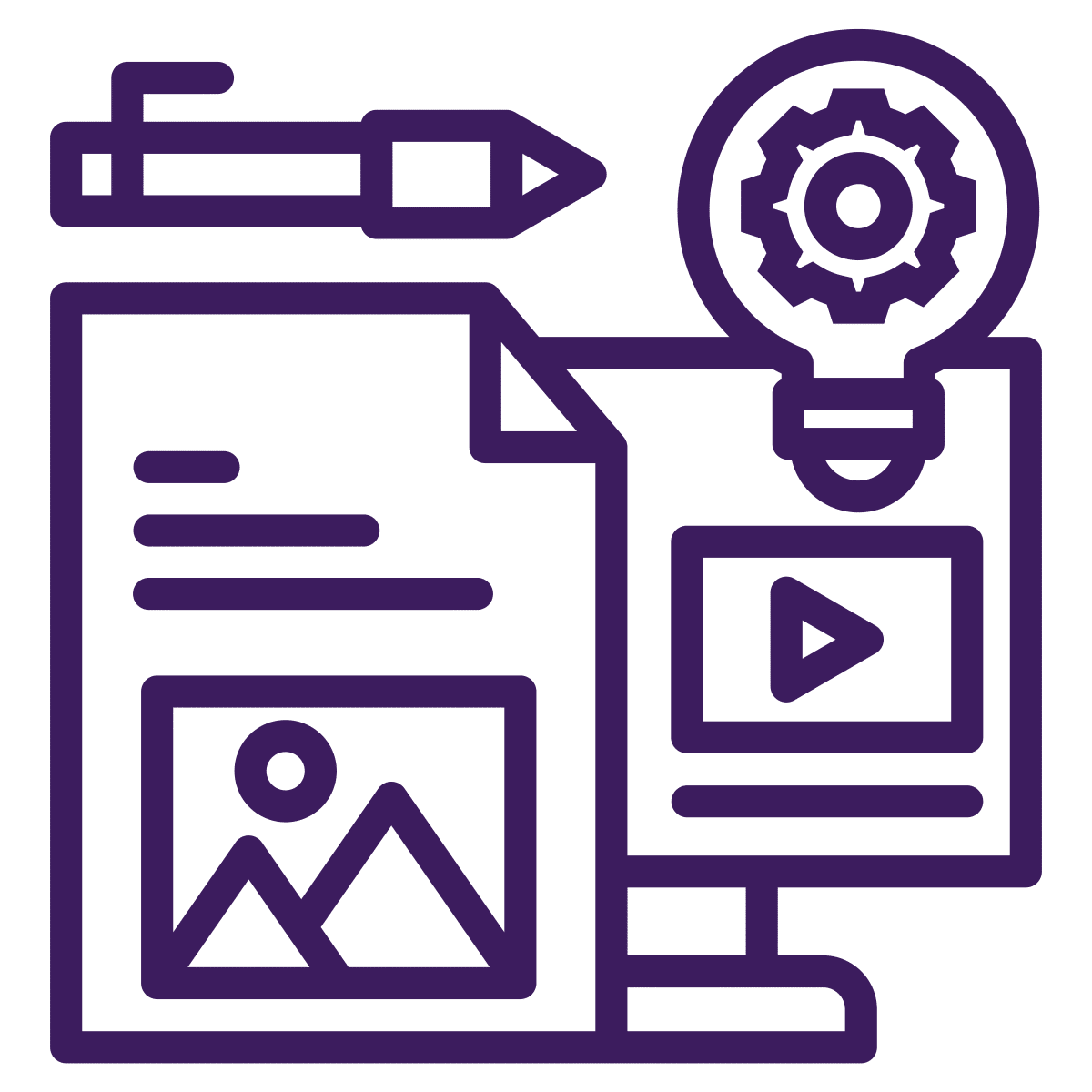
Once your organization’s knowledge domain is defined, the next step is to create the content models and content types that support that ontology, this is often referred to as content engineering.
Content types are the reusable templates that standardize the structure for a particular format of content such as articles, infographics, webinars, and product information, as well as the standard metadata that should be included with that content type (created date, author, department, related subjects, etc.).
If we think of Content Types as the cake pan in this analogy, a content model is the Cake Recipe. While the Content Type defines the structure of the content, the Content Model defines the meaning of that content. In the cake analogy, you may have a chocolate cake, a vanilla cake, and a carrot cake; theoretically, any of those recipes could be used in any of the pans. If the content type dictates how, the content model dictates what. In an organization this could look like a content model of a product that includes parts like the product title, the product value proposition, the product features, etc. This content model of a product could then be fit into many content types, such as a brochure, a web page, and an infographic. By creating content models and content types we give the AI model better insight into how the content is connected and the purpose it serves.
The structure of these templates provides AI with content in a consumable and semantically meaningful format where content sections and metadata are given to the AI model. A crucial part of content engineering is the creation of a taxonomy to describe the content. Taxonomies should be user-centric, highlighting users’ terminology to talk about content. The terms within a taxonomy and the associated synonyms improve an AI’s capability to utilize the content. Additionally, content types and content models facilitate the consistent display of information and configuration of advanced search features, improving the user experience when looking for and viewing content.
Componentize the Content
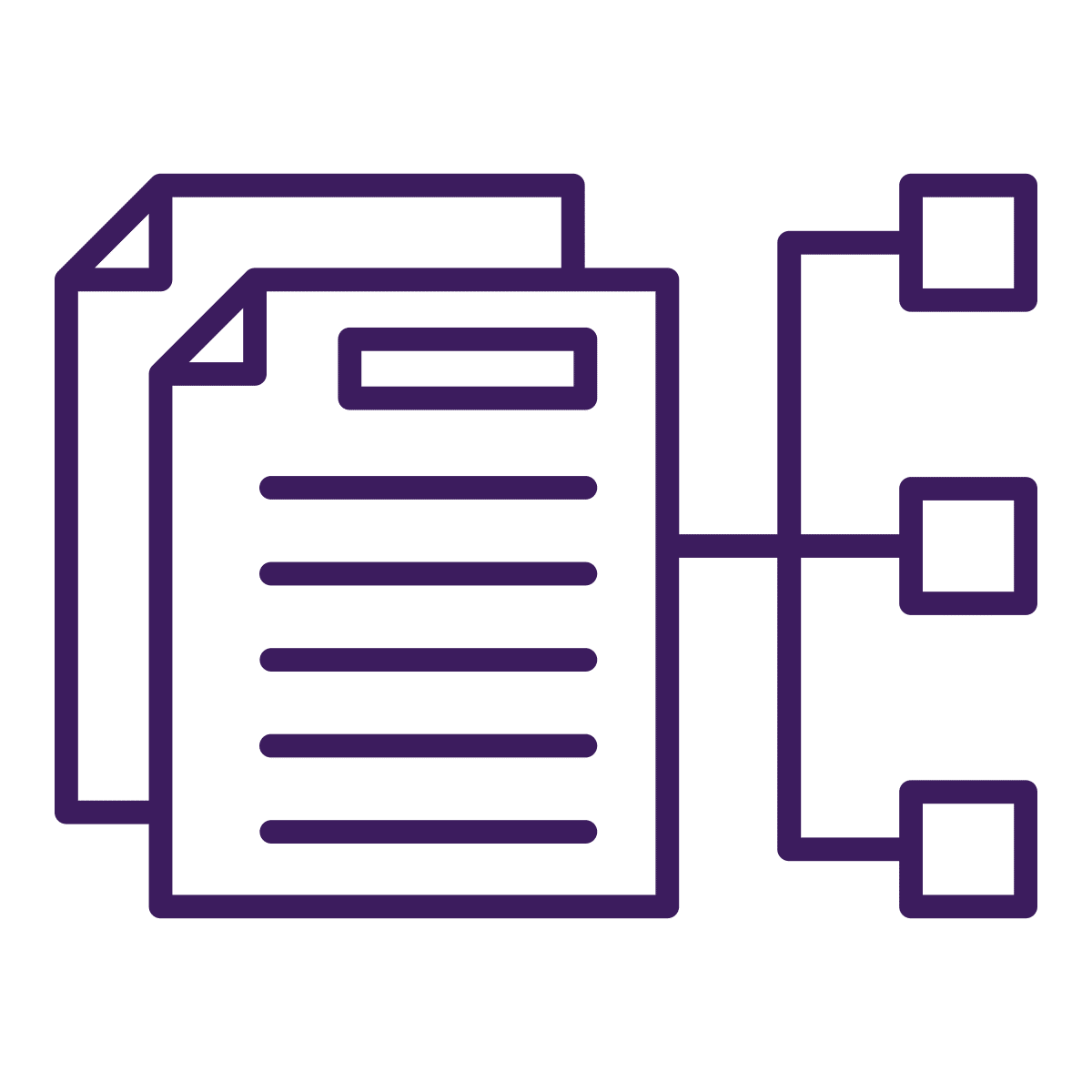
Once the content is structured and cleaned, a common next step is to break up the content into smaller sections according to the content model. This process has many names, such as content deconstruction, content chunking, or the creation of content components. In content deconstruction, structured content is split into smaller semantically meaningful sections. Each section or component has a standalone purpose, even without the context of the original document. Content components are often managed in a component content management system (CCMS), providing the following benefits:
Similar to the user benefits, content components provide AI with user-generated components of content as opposed to requiring the AI to perform statistical chunking. The content chunks allow an AI to identify relevant text inputs quickly and more accurately than if fed entire large documents.
Conclusion
Through effective content strategy, content audit, and content engineering, organizations can efficiently manage information and ensure that AI has correct, comprehensive content with semantically meaningful relationships. A well-defined content strategy provides a framework to curate old and new information, allowing organizations to continuously feed information into AI, keeping its internal models up-to-date. A well-structured content audit will ensure preparation time is spent on the areas that will make the most difference in AI-readiness such as structure, standardization, componentization, and relationships across content. Well-thought-out content engineering will enable content reuse and personalization at scale through machine readable structure.
Are you seeking help defining a content strategy, auditing your content for AI-readiness, or training your AI to understand your domain? Contact us and let us know how we can help!
Special thank you to James Midkiff for his contributions to the first draft of this blog post!