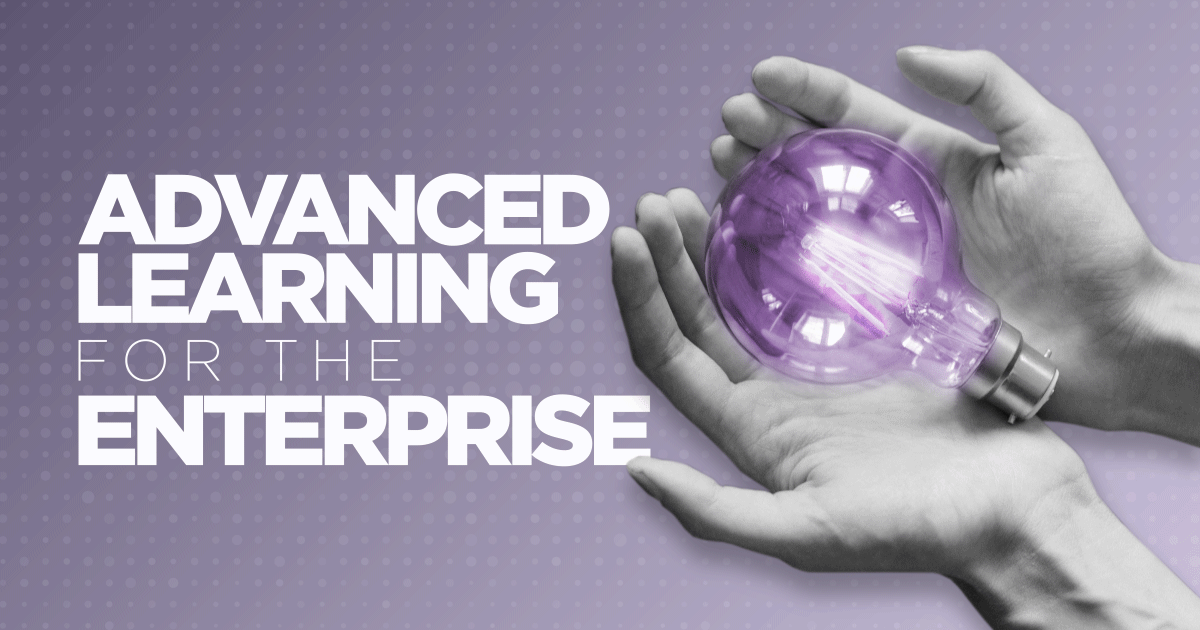
I’ve recently been expressing how pleased I am with the trend of Knowledge Management and Learning functions coming together. There’s a lot to like about this merger of two disciplines. At its core, KM should always serve as an enabling factor to effective learning and performance within an organization. KM can ensure the right (learning) content is current, available, and findable by the right people at the right time. KM can further ensure the right experts are recognized within the organization, available to upskill other employees, and fill in gaps in digital knowledge. Going even further, KM can help to structure and organize the wide array of learning assets that exist in a mature enterprise, moving well beyond simple course content to a vast learning ecosystem of content, people, and experiences that can improve learning and performance. In short, KM helps learners learn in the most efficient, complete, and flexible ways possible.
I’m now seeing a third element come into play, that being Enterprise Artificial Intelligence. When taxonomies, ontologies, componentized content, graph databases, and machine learning are brought into the spheres of KM and Learning, dream use cases suddenly come into focus as business reality. Each of these advanced learning use cases are no longer a dream for the distant future, but are instead achievable today. Indeed, we’ve successfully deployed each of the following at an enterprise scale for some of the world’s largest and most complex organizations.
The Top Four Advanced Learning Use Cases are:
- Automated Learning Path Assembly
- Aspirational Learning
- OmniLearning
- Adaptive Learning
Automated Learning Path Assembly
Learning Path Assembly, or Curriculum Assembly, is exactly what it sounds like. It enables the creation of learner-centric learning paths based on a wide array of factors, including their background, education, past experience, competencies, job goals, and job description. Too frequently, the learning community makes the mistake of assuming all people in a particular position are starting from the same place. With an automated learning path assembly solution, each individual learner’s starting place is factored into the design, ensuring that each individual employee is met where they are, to upskill the fastest and most successfully. This ensures the most advanced learners aren’t bored with background materials, while those who need more remedial support aren’t discouraged from the start and have an equal opportunity to thrive.
Imagine a scenario where an organization is onboarding thirty new analysts in a single class from various Masters programs. Though all are qualified for the job, they’ve come into the organization with vastly different backgrounds. Though all are expected to perform the same duties, they possess a different range of hard and soft skills to be successful. For most organizations, all thirty would be given the same onboarding, training, and development programs. Perhaps the lowest and highest initial performers would be given specific attention, but overall, due to the limitations of staff and resources, the one-size-fits-all approach would be employed. As a result, the class of thirty would likely see some quick attrition and underperformance.
With an automated learning path assembly solution, on the other hand, these thirty individuals would be treated as exactly that: individuals. Based on their starting points, skills, education, and experience, they’d each receive a fully customized onboarding to ensure they can perform their job from a position of confidence, quality, and consistency. Those lacking a key competency would have it addressed from the start to avoid performance issues that would be discouraging for them, and bad for the company’s performance. Meanwhile, those with a strong baseline of skills, capabilities, and experience would be moved through the learning process faster, ensuring they can be deployed to deliver for the organization as soon as they’re ready, instead of when the obligatory X hours of training are completed.
Learning path assembly also helps to address another major challenge in today’s leading organizations. The speed of business is increasing, and changes to operations sometimes come on a daily or weekly basis. With a dynamic assembly solution, the moment a specific learning component becomes outdated, it can be seamlessly swapped out for the new and current learning component.
Aspirational Learning
Expanding on the use case of automated learning path assembly, aspirational learning (also known as self-designated learning paths or self-directed learning paths) puts the learning journey and its destination in the hands of each individual learner. With aspirational learning, rather than the definition of done being defined by the organization, the individual learner is empowered to define their learning goals. The core concept of aspirational learning allows each individual learner to identify not only what they want to learn, but “who” they want to be within the organization. In some systems, we’ve even designed this to allow an individual to choose the position they wish to develop towards (as in, when I “grow up,” I wanted Joe’s job…teach me how to perform in it). This use case is most applicable for organizations with multiple career paths, but the core concept can be applied in a number of scenarios.
In the discipline of knowledge management, we talk about the distinction between the ability to find information and the ability to discover information:
- Findability: The ability to find the specific knowledge asset you’re searching for.
- Discoverability: The ability to discover knowledge assets you didn’t even know were there.
Aspirational learning allows each individual learner to discover not only what they want to learn, but what they want to be within the organization. At EK, we’ve even designed systems which allow an individual to choose the position they wish to develop towards (as in, when I “grow up,” I wanted Joe’s job…teach me how to perform in it). This use case is most applicable for organizations with multiple career paths, but the core concept can be applied in a number of scenarios.
In most organizations learning falls into one of three categories 1) the obligatory, box-checking learning, such as workplace safety or cyber-security, 2) the performant, focusing on how to do one’s job, and perhaps the job directly senior to it, and 3) the elective, which broadly includes all the wonderful, exciting, stretching elements that move someone beyond and even outside of their known, strict career path, potentially to great value to the individual and the organization for whom they work. In too many organizations, the focus is one the obligatory and the performant, but elective learning and development is where your best potential employees become your best actual employees.
With an aspirational learning solution, employees are given the resources to discover where they want to go in the organization and into whom they wish to develop. Armed with those options, each employee is set up to reach beyond their current state and capabilities and learn new skills, some which might directly serve their current position, with others that might serve the organization for years to come in new ways.
Notably, not all employees will complete these aspirational learning journeys, and still others will reset their path and change their course, perhaps several times over. Some of the most successful people I know changed their major in college, not to mention those who reinvented their careers years later. If organizations give their employees the ability to discover a future in their organization, retention will increase, but innovation and performance will as well.
OmniLearning
One of the most consistent problem statements I receive from leaders in learning and development is that their actual learning content is, to put it frankly, a mess. Global organizations tend to struggle with several problems:
- Their learning content is spread through several different and unlinked systems and repositories, meaning most potential learners (let alone learning professionals) struggle to find and leverage the full breadth of actual learning content available.
- The content is unstructured and unordered, meaning that it lacks the context to make it relatable to other pieces or content or mapping it to attributes like learner type or competency.
- The content itself lacks consistency in form, style, and tone, making the learning content disjointed, and forcing learners to navigate through different vocabularies and styles in order to accomplish their learning goals.
- The delivery mechanisms for learning content, be they Learning Management Systems, portals, or search experiences suffer from usability issues, lack of personalization to different learners and learner goals, and often present an incomplete view of what is actually available.
When all of this gets wrapped together, it means that learning professionals are often replicating work that has already been done, while the learners themselves are unable to obtain the full value of the learning materials. When you consider that learning content and learning assets are diversifying to include not just traditional training, but also job aids, performance support, access to experts, and community learning, the challenge of organizing and managing your learning assets is becoming even more complex.
OmniLearning helps to address this complexity by:
- Adding structure in the form of taxonomies and ontologies to all learning content, enabling mapping between content and learners;
- Providing a single location to find and access the learning content no matter where the content is stored;
- Integrating advanced content management systems in order to deconstruct learning content down to more easily consumable and flexible size, while also improving content structure and consistency, and
- Adding in customized user interfaces and multi-channel delivery.
Taken together, this means that OmniLearning gets all the right content to the right learners in a way they can easily understand and act upon.
Adaptive Learning
Finally, Adaptive Learning adds an additional level of customization and flexibility to learning journeys, once again recognizing that different learners learn at different paces and struggle with different concepts. Adaptive Learning integrates small checkpoints in the form of quizzes, small practical tests, and self-analysis in order to gauge whether the learner requires a different pace of learning, or more advanced or remedial content in particular areas. If a learner is excelling in a particular space, there’s no reason to cause frustration by forcing them to go through modules or review materials they’re already expert in. Whereas Adaptive Learning has historically been employed within a course, with today’s technology and standards, the same concept can be applied across each learner’s complete journey, between courses, and including all forms of learning assets.
Putting it All Together
What this all means, when put together in the right way, is that each individual learner will have greater control over their path, greater flexibility to learn in the ways they’ll be most successful, and a greater overall learning experience. The benefits for the organization are numerous, not just in greater use of learning resources and improved consistency and quality of learning materials, but expanded to hard returns on investment via higher employee retention and satisfaction, and improved cost avoidance with employees retaining more knowledge and better protecting the organization from risk in all its forms.
AI is making some incredibly complex KM and Learning use cases a reality. These are exciting times, where the scale of solvable problems has drastically increased, and the finesse with which said problems can be solved is increasing as well.